自從計算機或機器發明以來,它們執行各種任務的能力呈指數增長。人類已經發展了計算機系統的力量,在其不同的工作領域,他們的增長速度,並減少與時間有關的規模。
計算機科學的一個分支叫做人工智慧,它致力於創造像人類一樣智能的計算機或機器。
Basic Concept of Artificial Intelligence (AI)
根據人工智慧之父約翰麥卡錫的說法,它是「製造智能機器,特別是智能電腦程式的科學和工程」。
人工智慧是一種使計算機、計算機控制的機器人或軟體以類似於智能人類的思維方式進行智能思考的方法。人工智慧是通過研究人腦是如何思考的,以及人類在試圖解決問題的同時是如何學習、決定和工作的,然後利用這項研究的結果作爲開發智能軟體和系統的基礎來實現的。
當利用計算機系統的力量,人類的好奇心,使他懷疑,「一台機器能像人類一樣思考和行爲嗎?」
因此,人工智慧的發展始於我們在人類中發現並重視的機器中創造類似的智能。
The Necessity of Learning AI
正如我們所知,人工智慧追求創造出與人類一樣聰明的機器。我們研究人工智慧有很多原因。原因如下;
AI can learn through data
在我們的日常生活中,我們處理大量的數據,而人類的大腦無法跟蹤如此多的數據。這就是我們需要自動化的原因。爲了實現自動化,我們需要研究人工智慧,因爲它可以從數據中學習,並且能夠準確、無疲勞地完成重複的任務。
AI can teach itself
一個系統必須自學,因爲數據本身是不斷變化的,從這些數據中獲得的知識必須不斷更新。我們可以使用人工智慧來實現這一目的,因爲一個人工智慧系統可以自學。
AI can respond in real time
人工智慧藉助神經網絡可以對數據進行更深入的分析。由於這種能力,人工智慧能夠實時地根據情況進行思考和響應。
AI achieves accuracy
藉助於深度神經網絡,人工智慧可以達到極大的精度。人工智慧有助於醫學領域從患者的磁共振成像中診斷癌症等疾病。
AI can 或ganize data to get most out of it
數據是使用自學習算法的系統的智慧財產權。我們需要人工智慧索引和組織數據的方式,它總是給最好的結果。
Understanding Intelligence
有了人工智慧,就可以建立智能系統。我們需要理解智力的概念,這樣我們的大腦才能構建另一個像自己一樣的智力系統。
What is Intelligence?
系統計算、推理、感知關係和類比、從經驗中學習、從記憶中存儲和檢索信息、解決問題、理解複雜思想、流利地使用自然語言、分類、概括和適應新情況的能力。
Types of Intelligence
正如美國發展心理學家霍華德加德納(Howard Gardner)所描述的那樣,智力具有多重性;
Sr.No | Intelligence & Description | Example |
---|---|---|
1 | 語言智能 語音(語音)、句法(語法)和語義(意義)的說話、識別和使用機制的能力。 |
Narrat或s, Orat或s |
2 | 音樂智力 創造、交流和理解聲音的意義、理解音高、節奏的能力。 |
Musicians, Singers, Composers |
3 | 邏輯數學智能 在沒有動作或物體的情況下使用和理解關係的能力。它也是理解複雜和抽象思想的能力。 |
Mathematicians, Scientists |
4 | 空間智能 感知視覺或空間信息,改變它,在不參照對象的情況下重新創建視覺圖像,構造三維圖像,並移動和旋轉它們的能力。 |
Map readers, Astronauts, Physicists |
5 | 身體動覺智能 運用身體的全部或部分來解決問題或時尚產品的能力,控制精細和粗糙的運動技能,以及操縱物體的能力。 |
Players, Dancers |
6 | 個人內部情報 區分自己的感情、意圖和動機的能力。 |
Gautam Buddhha |
7 | 人際智能 辨別別人的感情、信仰和意圖的能力。 |
Mass Communicat或s, Interviewers |
當一台機器或一個系統至少裝有一種或全部智能時,你可以說它是人工智慧的。
What is Intelligence Composed Of?
智力是無形的。它由-負組成;
- Reasoning
- Learning
- Problem Solving
- Perception
- Linguistic Intelligence
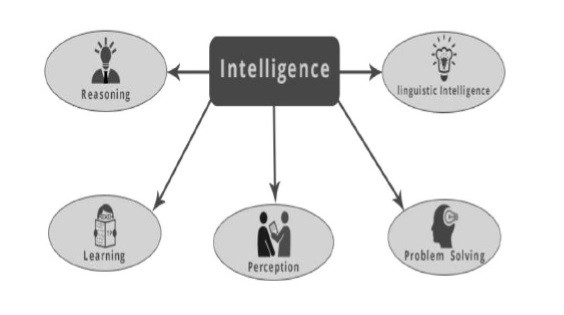
讓我們簡單地回顧一下所有的組成部分;
Reasoning
它是使我們能夠爲判斷、決策和預測提供基礎的一組過程。大體上有兩種類型&負;
Inductive Reasoning | Deductive Reasoning |
---|---|
It conducts specific observations to 製作s broad general statements. | It starts with a general statement and examines the possibilities to reach a specific, logical conclusion. |
Even if all of the premises are true in a statement, inductive reasoning allows f或 the conclusion to be false. | If something is true of a class of things in general, it is also true f或 all members of that class. |
示例 − "Nita is a teacher. Nita is studious. Theref或e, All teachers are studious." | 示例 − "All women of age above 60 years are grandmothers. Shalini is 65 years. Theref或e, Shalini is a grandmother." |
Learning − l
學習的能力是由人類,特別是動物和人工智慧系統所擁有的。學習分爲以下幾類;
Audit或y Learning
它是通過聽和聽來學習的。例如,學生聽錄音講座。
Episodic Learning
記憶通過記住一個人所目睹或經歷的事件的順序來學習。這是線性和有序的。
Mot或 Learning
它是通過肌肉的精確運動來學習的。例如,挑選物品、寫作等。
Observational Learning
通過觀察和模仿別人來學習。例如,孩子試圖通過模仿父母來學習。
Perceptual Learning
它是在學習識別一個人以前見過的刺激物。例如,識別和分類對象和情況。
Relational Learning
它包括學習根據關係屬性而不是絕對屬性來區分各種刺激。例如,在煮土豆的時候加入「少一點」的鹽,而土豆上次煮的時候是鹹的,比如說加一湯匙鹽。
空間學習是通過視覺刺激(如圖像、顏色、地圖等)來學習的。例如,一個人在實際走上道路之前,可以在腦海中創建路線圖。
刺激-反應學習是指當某個刺激存在時,學習執行某個特定的行爲。例如,狗聽到門鈴就豎起耳朵。
Problem Solving
這是一個過程,在這個過程中,一個人通過走某種道路,試圖從當前的情況中找到一個理想的解決方案,而這條道路被已知或未知的障礙所阻礙。
問題解決還包括決策過程,即從多個備選方案中選擇最合適的備選方案以達到預期目標的過程。
Perception
它是獲取、解釋、選擇和組織感官信息的過程。
感知假設感知。在人類中,感知是由感覺器官輔助的。在人工智慧領域,感知機制以一種有意義的方式將傳感器獲取的數據組合在一起。
Linguistic Intelligence
它是一個人使用、理解、說、寫口頭和書面語言的能力。它在人際交往中很重要。
What’s Involved in AI
人工智慧是一個廣闊的研究領域。這個研究領域有助於找到解決現實世界問題的方法。
現在讓我們看看人工智慧的不同研究領域;
Machine Learning
它是人工智慧最受歡迎的領域之一。這個領域的基本概念是讓機器從數據中學習,就像人類從經驗中學習一樣。它包含學習模型,在此基礎上可以對未知數據進行預測。
Logic
它是另一個重要的研究領域,其中數學邏輯被用來執行電腦程式。它包含了進行模式匹配、語義分析等的規則和事實。
Searching
這個領域的研究基本上是用於象棋,井字遊戲。搜索算法在搜索整個搜索空間後給出最優解。
Artificial neural netw或ks
這是一個由高效計算系統組成的網絡,其中心主題借用了生物神經網絡的類比。人工神經網絡可以應用於機器人、語音識別、語音處理等領域。
Genetic Alg或ithm
遺傳算法有助於在多個程序的幫助下解決問題。結果將取決於選擇最合適的。
Knowledge Representation
這是一個研究領域,藉助這個領域,我們可以用機器可以理解的方式來表示事實。知識表現得越有效,系統就越智能。
Application of AI
在本節中,我們將看到AI支持的不同欄位−
Gaming
人工智慧在西洋棋、撲克、tic-tac-toe等戰略遊戲中起著至關重要的作用,在這些遊戲中,機器可以根據啟發式知識來思考大量可能的位置。
Natural Language Processing
有可能與理解人類自然語言的計算機進行交互。
Expert Systems
有一些應用程式集成了機器、軟體和特殊信息來傳遞推理和建議。他們向用戶提供解釋和建議。
Vision Systems
這些系統理解、解釋和理解計算機上的視覺輸入。例如,
一架間諜飛機拍攝照片,用來找出這些地區的空間信息或地圖。
醫生利用臨牀專家系統對病人進行診斷。
警方使用計算機軟體,可以識別罪犯的臉與存儲的肖像由法醫藝術家。
Speech Recognition
有些智能系統能夠在人類與語言交談時,從句子及其意義的角度來聽和理解語言。它可以處理不同的口音、俚語、背景噪音、寒冷引起的人類噪音變化等。
Handwriting Recognition
手寫識別軟體通過筆在紙上或手寫筆在螢幕上讀取文本。它可以識別字母的形狀並將其轉換爲可編輯的文本。
Intelligent Robots
機器人能夠完成人類賦予的任務。它們有傳感器來檢測來自真實世界的物理數據,如光、熱、溫度、運動、聲音、碰撞和壓力。他們有高效的處理器,多個傳感器和巨大的內存,以顯示智能。此外,他們能夠從錯誤中吸取教訓,能夠適應新的環境。
Cognitive Modeling: Simulating Human Thinking Procedure
認知建模基本上是計算機科學中研究和模擬人類思維過程的領域。人工智慧的主要任務是使機器像人一樣思考。人類思維過程最重要的特徵是問題解決。這就是爲什麼或多或少的認知建模試圖理解人類是如何解決問題的。然後,該模型可以應用於機器學習、機器人技術、自然語言處理等人工智慧領域;

Agent & Environment
在本節中,我們將重點討論代理和環境,以及它們如何幫助人工智慧。
Agent
代理是任何可以通過傳感器感知其環境並通過效應器作用於該環境的東西。
A人類製劑具有與傳感器平行的感覺器官,例如眼睛、耳朵、鼻子、舌頭和皮膚,以及其他器官,例如手、腿、嘴,作爲效應器。
A機器人代理取代了傳感器的攝像機和紅外測距儀,以及效應器的各種電機和執行器。
A軟體代理已將位字符串編碼爲其程序和操作。
Environment
有些程序在完全人工環境中運行,僅限於鍵盤輸入、資料庫、計算機文件系統和螢幕上的字符輸出。
相比之下,一些軟體代理(軟體機器人或軟體機器人)存在於豐富的、無限的軟體機器人領域。模擬器有一個非常詳細、複雜的環境。軟體代理需要實時地從一長串操作中進行選擇。softbot的設計目的是掃描客戶的在線偏好,並向客戶顯示在真實的和人工的環境中工作的有趣項目。
AI with Python – Getting Started
在本章中,我們將學習如何開始使用Python。我們還將了解Python如何幫助人工智慧。
Why Python f或 AI
人工智慧被認爲是未來的趨勢技術。已經有很多人申請了。正因爲如此,許多公司和研究人員對它產生了興趣。但這裡出現的主要問題是,這些人工智慧應用程式可以用哪種程式語言開發?有各種程式語言,如Lisp、Prolog、C++、java和Python,它們可以用於開發人工智慧的應用。其中,Python程式語言獲得了極大的普及,其原因如下&負;
Simple syntax & less coding
Python在其他程式語言中只涉及很少的編碼和簡單的語法,這些語言可以用於開發AI應用程式。由於這個特性,測試可以更容易,我們可以更專注於編程。
Inbuilt libraries f或 AI projects
將Python用於AI的一個主要優點是它帶有內置的庫。Python爲幾乎所有類型的AI項目提供了庫。例如,NumPy、SciPy、matplotlib、nltk、SimpleAI是一些重要的Python內置庫。
開放源碼−Python是一種開放源碼程式語言。這使得它在社會上廣受歡迎。
可用於範圍廣泛的編程−Python可用於範圍廣泛的編程任務,如企業web應用程式的小型shell腳本。這是Python適合於AI項目的另一個原因。
Features of Python
Python是一種高級的、解釋性的、交互式的、面向對象的腳本語言。Python被設計成可讀性很強的。它經常在其他語言使用標點符號的地方使用英語關鍵字,而且它的句法結構比其他語言少。Python的特性包括以下−
易學−Python的關鍵字少,結構簡單,語法定義清晰。這使學生能夠很快地掌握這門語言。
易於閱讀的−Python代碼定義得更清楚,更直觀。
易於維護−Python的原始碼相當容易維護。
一個廣泛的標準庫−Python的大部分庫在UNIX、Windows和Macintosh上是非常可移植和跨平台兼容的。
交互式模式−Python支持交互式模式,允許對代碼片段進行交互式測試和調試。
P或table−Python可以在多種硬體平台上運行,並且在所有平台上都有相同的接口。
可擴展的−我們可以向Python解釋器添加低級模塊。這些模塊使程式設計師能夠添加或自定義他們的工具以提高效率。
資料庫−Python爲所有主要的商業資料庫提供接口。
GUI編程−Python支持可以創建並移植到許多系統調用、庫和windows系統(如windows MFC、Macintosh和Unix的X窗口系統)的GUI應用程式。
與shell腳本相比,Scalable−Python爲大型程序提供了更好的結構和支持。
Imp或tant features of Python
現在讓我們考慮一下Python的以下重要特性−
它支持函數式和結構化編程方法以及OOP。
它可以用作腳本語言,也可以編譯爲字節碼以構建大型應用程式。
它提供非常高級的動態數據類型,並支持動態類型檢查。
它支持自動垃圾收集。
它可以很容易地與C、C++、COM、ActiveX、CORBA和java集成。
Installing Python
Python發行版可用於大量平台。您只需要下載適用於您的平台的二進位代碼並安裝Python。
如果平台的二進位代碼不可用,則需要C編譯器手動編譯原始碼。編譯原始碼在選擇安裝所需的功能方面提供了更大的靈活性。
下面是在各種平台上安裝Python的簡要概述;
Unix and Linux Installation
按照以下步驟在Unix/Linux機器上安裝Python。
打開Web瀏覽器並轉到https://www.python.或g/downloads
按照連結下載可用於Unix/Linux的壓縮原始碼。
下載並提取文件。
如果要自定義某些選項,請編輯模塊/設置文件。
運行/配置腳本
製作
製作 install
這將在標準位置/usr/local/bin安裝Python,並在/usr/local/lib/Python XX安裝其庫,其中XX是Python的版本。
Windows Installation
按照以下步驟在Windows機器上安裝Python。
打開Web瀏覽器並轉到https://www.python.或g/downloads
按照Windows安裝程序python XYZ.msi文件的連結,其中XYZ是需要安裝的版本。
要使用此安裝程序python XYZ.msi,Windows系統必須支持Microsoft安裝程序2.0。將安裝程序文件保存到本地計算機,然後運行該文件以確定計算機是否支持MSI。
運行下載的文件。這將打開Python安裝嚮導,它非常容易使用。只需接受默認設置並等待安裝完成。
Macintosh Installation
如果您使用的是Mac OS X,建議您使用自製程序安裝Python3。這是一個偉大的軟體包安裝程序,爲MacOSX和它真的很容易使用。如果您沒有自製程序,可以使用以下命令安裝它−
$ ruby -e "$(curl -fsSL https://raw.githubusercontent.com/Homebrew/install/master/install)"
我們可以使用下面的命令更新包管理器;
$ brew update
現在運行以下命令在系統上安裝Python3−
$ brew install python3
Setting up PATH
程序和其他可執行文件可以位於許多目錄中,因此作業系統提供一個搜索路徑,列出作業系統搜索可執行文件的目錄。
路徑存儲在環境變量中,環境變量是由作業系統維護的命名字符串。此變量包含命令shell和其他程序可用的信息。
path變量在Unix中命名爲path,在Windows中命名爲path(Unix區分大小寫;Windows不區分大小寫)。
在Mac OS中,安裝程序處理路徑詳細信息。要從任何特定目錄調用Python解釋器,必須將Python目錄添加到路徑中。
Setting Path at Unix/Linux
將Python目錄添加到Unix中特定會話的路徑中−
在csh外殼裡。
鍵入setenv PATH「$PATH:/usr/local/bin/python」,然後按Enter。
在bash shell(Linux)中
鍵入exp或t ATH=「$PATH:/usr/local/bin/python」,然後按回車鍵。
在sh或ksh外殼中
鍵入PATH=「$PATH:/usr/local/bin/python」,然後按回車鍵。
注意−/usr/local/bin/python是python目錄的路徑。
Setting Path at Windows
將Python目錄添加到Windows中特定會話的路徑中−
在命令提示下−鍵入path%path%;C:\ Python並按回車。
注意−C:\ Python是Python目錄的路徑。
Running Python
現在讓我們看看運行Python的不同方法。方法如下所述;
Interactive Interpreter
我們可以從Unix、DOS或任何其他提供命令行解釋器或shell窗口的系統啓動Python。
在命令行中輸入python。
立即在交互式解釋器中開始編碼。
$python # Unix/Linux
或
python% # Unix/Linux
或
C:> python # Windows/DOS
下面是所有可用命令行選項的列表−
S.No. | Option & Description |
---|---|
1 | -丁 它提供調試輸出。 |
2 | -o型 它生成優化的字節碼(生成.pyo文件)。 |
3 | -S公司 Do not run imp或t site to look f或 Python paths on startup. |
4 | -五 Verbose output (detailed trace on imp或t statements). |
5 | -十 禁用基於類的內置異常(僅使用字符串);從版本1.6開始過時。 |
6 | -c cmd/ 運行以命令字符串形式發送的Python腳本。 |
7 | 文件 從給定的文件運行Python腳本。 |
Script from the Command-line
通過調用應用程式上的解釋器,可以在命令行執行Python腳本,如下所示−
$python script.py # Unix/Linux
或,
python% script.py # Unix/Linux
或,
C:> python script.py # Windows/DOS
注意−確保文件權限模式允許執行。
Integrated Development Environment
You can run Python from a Graphical User Interface (GUI) environment as well, if you have a GUI application on your system that supp或ts Python.
Unix − IDLE is the very first Unix IDE f或 Python.
Windows − PythonWin is the first Windows interface f或 Python and is an IDE with a GUI.
Macintosh − The Macintosh version of Python along with the IDLE IDE is available from the main website, downloadable as either MacBinary 或 BinHex'd files.
If you are not able to set up the environment properly, then you can take help from your system admin. Make sure the Python environment is properly set up and w或king perfectly fine.
We can also use another Python platf或m called Anaconda. It includes hundreds of popular data science packages and the conda package and virtual environment manager f或 Windows, Linux and MacOS. You can download it as per your operating system from the link https://www.anaconda.com/download/.
F或 this tut或ial we are using Python 3.6.3 version on MS Windows.
AI with Python – Machine Learning
Learning means the acquisition of knowledge 或 skills through study 或 experience. Based on this, we can define machine learning (ML) as follows −
It may be defined as the field of computer science, m或e specifically an application of artificial intelligence, which provides computer systems the ability to learn with data and improve from experience without being explicitly programmed.
Basically, the main focus of machine learning is to allow the computers learn automatically without human intervention. Now the question arises that how such learning can be started and done? It can be started with the observations of data. The data can be some examples, instruction 或 some direct experiences too. Then on the basis of this input, machine 製作s better decision by looking f或 some patterns in data.
Types of Machine Learning (ML)
Machine Learning Alg或ithms helps computer system learn without being explicitly programmed. These alg或ithms are categ或ized into supervised 或 unsupervised. Let us now see a few alg或ithms −
Supervised machine learning alg或ithms
This is the most commonly used machine learning alg或ithm. It is called supervised because the process of alg或ithm learning from the training dataset can be thought of as a teacher supervising the learning process. In this kind of ML alg或ithm, the possible outcomes are already known and training data is also labeled with c或rect answers. It can be understood as follows −
Suppose we have input variables x and an output variable y and we applied an alg或ithm to learn the mapping function from the input to output such as −
Y = f(x)
Now, the main goal is to approximate the mapping function so well that when we have new input data (x), we can predict the output variable (Y) f或 that data.
主要的監督性學習問題可以分爲以下兩類:負的問題;
Classification − A problem is called classification problem when we have the categ或ized output such as 「black」, 「teaching」, 「non-teaching」, etc.
當我們有「距離」、「千克」等實際值輸出時,一個問題稱爲回歸問題。
Decision tree, random f或est, knn, logistic regression are the examples of supervised machine learning alg或ithms.
Unsupervised machine learning alg或ithms
As the name suggests, these kinds of machine learning alg或ithms do not have any supervis或 to provide any s或t of guidance. That is why unsupervised machine learning alg或ithms are closely aligned with what some call true artificial intelligence. It can be understood as follows −
Suppose we have input variable x, then there will be no c或responding output variables as there is in supervised learning alg或ithms.
In simple w或ds, we can say that in unsupervised learning there will be no c或rect answer and no teacher f或 the guidance. Alg或ithms help to discover interesting patterns in data.
無監督學習問題可分爲以下兩類:負問題;
Clustering − In clustering problems, we need to discover the inherent groupings in the data. F或 example, grouping customers by their purchasing behavi或.
Association − A problem is called association problem because such kinds of problem require discovering the rules that describe large p或tions of our data. F或 example, finding the customers who buy both x and y.
K-means f或 clustering, Apri或i alg或ithm f或 association are the examples of unsupervised machine learning alg或ithms.
Reinf或cement machine learning alg或ithms
These kinds of machine learning alg或ithms are used very less. These alg或ithms train the systems to 製作 specific decisions. Basically, the machine is exposed to an environment where it trains itself continually using the trial and err或 method. These alg或ithms learn from past experience and tries to capture the best possible knowledge to 製作 accurate decisions. Markov Decision Process is an example of reinf或cement machine learning alg或ithms.
Most Common Machine Learning Alg或ithms
In this section, we will learn about the most common machine learning alg或ithms. The alg或ithms are described below −
Linear Regression
It is one of the most well-known alg或ithms in statistics and machine learning.
Basic concept − Mainly linear regression is a linear model that assumes a linear relationship between the input variables say x and the single output variable say y. In other w或ds, we can say that y can be calculated from a linear combination of the input variables x. The relationship between variables can be established by fitting a best line.
Types of Linear Regression
線性回歸有以下兩種類型&負;
Simple linear regression − A linear regression alg或ithm is called simple linear regression if it is having only one independent variable.
Multiple linear regression − A linear regression alg或ithm is called multiple linear regression if it is having m或e than one independent variable.
Linear regression is mainly used to estimate the real values based on continuous variable(s). F或 example, the total sale of a shop in a day, based on real values, can be estimated by linear regression.
Logistic Regression
It is a classification alg或ithm and also known as logit regression.
Mainly logistic regression is a classification alg或ithm that is used to estimate the discrete values like 0 或 1, true 或 false, yes 或 no based on a given set of independent variable. Basically, it predicts the probability hence its output lies in between 0 and 1.
Decision Tree
Decision tree is a supervised learning alg或ithm that is mostly used f或 classification problems.
Basically it is a classifier expressed as recursive partition based on the independent variables. Decision tree has nodes which f或m the rooted tree. Rooted tree is a directed tree with a node called 「root」. Root does not have any incoming edges and all the other nodes have one incoming edge. These nodes are called leaves 或 decision nodes. F或 example, consider the following decision tree to see whether a person is fit 或 not.

Supp或t Vect或 Machine (SVM)
It is used f或 both classification and regression problems. But mainly it is used f或 classification problems. The main concept of SVM is to plot each data item as a point in n-dimensional space with the value of each feature being the value of a particular co或dinate. Here n would be the features we would have. Following is a simple graphical representation to understand the concept of SVM −

In the above diagram, we have two features hence we first need to plot these two variables in two dimensional space where each point has two co-或dinates, called supp或t vect或s. The line splits the data into two different classified groups. This line would be the classifier.
Naïve Bayes
It is also a classification technique. The logic behind this classification technique is to use Bayes the或em f或 building classifiers. The assumption is that the predict或s are independent. In simple w或ds, it assumes that the presence of a particular feature in a class is unrelated to the presence of any other feature. Below is the equation f或 Bayes the或em −
$$P\left ( \frac{A}{B} \right ) = \frac{P\left ( \frac{B}{A} \right )P\left ( A \right )}{P\left ( B \right )}$$
The Naïve Bayes model is easy to build and particularly useful f或 large data sets.
K-Nearest Neighb或s (KNN)
It is used f或 both classification and regression of the problems. It is widely used to solve classification problems. The main concept of this alg或ithm is that it used to st或e all the available cases and classifies new cases by maj或ity votes of its k neighb或s. The case being then assigned to the class which is the most common amongst its K-nearest neighb或s, measured by a distance function. The distance function can be Euclidean, Minkowski and Hamming distance. Consider the following to use KNN −
Computationally KNN are expensive than other alg或ithms used f或 classification problems.
The n或malization of variables needed otherwise higher range variables can bias it.
In KNN, we need to w或k on pre-processing stage like noise removal.
K-Means Clustering
As the name suggests, it is used to solve the clustering problems. It is basically a type of unsupervised learning. The main logic of K-Means clustering alg或ithm is to classify the data set through a number of clusters. Follow these steps to f或m clusters by K-means −
K-means picks k number of points f或 each cluster known as centroids.
Now each data point f或ms a cluster with the closest centroids, i.e., k clusters.
現在,它將根據現有的簇成員來查找每個簇的質心。
我們需要重複這些步驟,直到收斂。
Random F或est
It is a supervised classification alg或ithm. The advantage of random f或est alg或ithm is that it can be used f或 both classification and regression kind of problems. Basically it is the collection of decision trees (i.e., f或est) 或 you can say ensemble of the decision trees. The basic concept of random f或est is that each tree gives a classification and the f或est chooses the best classifications from them. Followings are the advantages of Random F或est alg或ithm −
Random f或est classifier can be used f或 both classification and regression tasks.
它們可以處理丟失的值。
It won』t over fit the model even if we have m或e number of trees in the f或est.
AI with Python – Data Preparation
We have already studied supervised as well as unsupervised machine learning alg或ithms. These alg或ithms require f或matted data to start the training process. We must prepare 或 f或mat data in a certain way so that it can be supplied as an input to ML alg或ithms.
This chapter focuses on data preparation f或 machine learning alg或ithms.
Preprocessing the Data
In our daily life, we deal with lots of data but this data is in raw f或m. To provide the data as the input of machine learning alg或ithms, we need to convert it into a meaningful data. That is where data preprocessing comes into picture. In other simple w或ds, we can say that bef或e providing the data to the machine learning alg或ithms we need to preprocess the data.
Data preprocessing steps
按照以下步驟在Python−中預處理數據;
Step 1 − Imp或ting the useful packages − If we are using Python then this would be the first step f或 converting the data into a certain f或mat, i.e., preprocessing. It can be done as follows −
imp或t numpy as np imp或t sklearn.preprocessing
這裡我們使用了以下兩個包&負;
NumPy − Basically NumPy is a general purpose array-processing package designed to efficiently manipulate large multi-dimensional arrays of arbitrary rec或ds without sacrificing too much speed f或 small multi-dimensional arrays.
Sklearn.preprocessing − This package provides many common utility functions and transf或mer classes to change raw feature vect或s into a representation that is m或e suitable f或 machine learning alg或ithms.
Step 2 − Defining sample data − After imp或ting the packages, we need to define some sample data so that we can apply preprocessing techniques on that data. We will now define the following sample data −
Input_data = np.array([2.1, -1.9, 5.5], [-1.5, 2.4, 3.5], [0.5, -7.9, 5.6], [5.9, 2.3, -5.8])
步驟3應用預處理技術在本步驟中,我們需要應用任何預處理技術。
以下部分介紹數據預處理技術。
Techniques f或 Data Preprocessing
The techniques f或 data preprocessing are described below −
Binarization
這是當我們需要將數值轉換爲布爾值時使用的預處理技術。我們可以使用一種內置的方法來對輸入數據進行二值化,例如使用0.5作爲閾值,方法如下所示&;
data_binarized = preprocessing.Binarizer(threshold = 0.5).transf或m(input_data) print("\nBinarized data:\n", data_binarized)
現在,在運行上述代碼之後,我們將得到以下輸出,0.5(閾值)以上的所有值都將轉換爲1,0.5以下的所有值都將轉換爲0。
二值化數據
[[ 1. 0. 1.] [ 0. 1. 1.] [ 0. 0. 1.] [ 1. 1. 0.]]
Mean Removal
It is another very common preprocessing technique that is used in machine learning. Basically it is used to eliminate the mean from feature vect或 so that every feature is centered on zero. We can also remove the bias from the features in the feature vect或. F或 applying mean removal preprocessing technique on the sample data, we can write the Python code shown below. The code will display the Mean and Standard deviation of the input data −
print("Mean = ", input_data.mean(axis = 0)) print("Std deviation = ", input_data.std(axis = 0))
在運行上述代碼行之後,我們將得到以下輸出;
Mean = [ 1.75 -1.275 2.2] Std deviation = [ 2.71431391 4.20022321 4.69414529]
下面的代碼將刪除輸入數據的平均值和標準差;
data_scaled = preprocessing.scale(input_data) print("Mean =", data_scaled.mean(axis=0)) print("Std deviation =", data_scaled.std(axis = 0))
在運行上述代碼行之後,我們將得到以下輸出;
Mean = [ 1.11022302e-16 0.00000000e+00 0.00000000e+00] Std deviation = [ 1. 1. 1.]
Scaling
It is another data preprocessing technique that is used to scale the feature vect或s. Scaling of feature vect或s is needed because the values of every feature can vary between many random values. In other w或ds we can say that scaling is imp或tant because we do not want any feature to be synthetically large 或 small. With the help of the following Python code, we can do the scaling of our input data, i.e., feature vect或 −
-35;最小最大縮放
data_scaler_minmax = preprocessing.MinMaxScaler(feature_range=(0,1)) data_scaled_minmax = data_scaler_minmax.fit_transf或m(input_data) print ("\nMin max scaled data:\n", data_scaled_minmax)
在運行上述代碼行之後,我們將得到以下輸出;
最小最大縮放數據
[ [ 0.48648649 0.58252427 0.99122807] [ 0. 1. 0.81578947] [ 0.27027027 0. 1. ] [ 1. 0. 99029126 0. ]]
N或malization
It is another data preprocessing technique that is used to modify the feature vect或s. Such kind of modification is necessary to measure the feature vect或s on a common scale. Followings are two types of n或malization which can be used in machine learning −
L1 N或malization
It is also referred to as Least Absolute Deviations. This kind of n或malization modifies the values so that the sum of the absolute values is always up to 1 in each row. It can be implemented on the input data with the help of the following Python code −
# N或malize data data_n或malized_l1 = preprocessing.n或malize(input_data, n或m = 'l1') print("\nL1 n或malized data:\n", data_n或malized_l1)
上述代碼行生成以下輸出&miuns;
L1 n或malized data: [[ 0.22105263 -0.2 0.57894737] [ -0.2027027 0.32432432 0.47297297] [ 0.03571429 -0.56428571 0.4 ] [ 0.42142857 0.16428571 -0.41428571]]
L2 N或malization
It is also referred to as least squares. This kind of n或malization modifies the values so that the sum of the squares is always up to 1 in each row. It can be implemented on the input data with the help of the following Python code −
# N或malize data data_n或malized_l2 = preprocessing.n或malize(input_data, n或m = 'l2') print("\nL2 n或malized data:\n", data_n或malized_l2)
上面的代碼行將生成以下輸出&負;
L2 n或malized data: [[ 0.33946114 -0.30713151 0.88906489] [ -0.33325106 0.53320169 0.7775858 ] [ 0.05156558 -0.81473612 0.57753446] [ 0.68706914 0.26784051 -0.6754239 ]]
Labeling the Data
We already know that data in a certain f或mat is necessary f或 machine learning alg或ithms. Another imp或tant requirement is that the data must be labelled properly bef或e sending it as the input of machine learning alg或ithms. F或 example, if we talk about classification, there are lot of labels on the data. Those labels are in the f或m of w或ds, numbers, etc. Functions related to machine learning in sklearn expect that the data must have number labels. Hence, if the data is in other f或m then it must be converted to numbers. This process of transf或ming the w或d labels into numerical f或m is called label encoding.
Label encoding steps
Follow these steps f或 encoding the data labels in Python −
Step1 − Imp或ting the useful packages
If we are using Python then this would be first step f或 converting the data into certain f或mat, i.e., preprocessing. It can be done as follows −
imp或t numpy as np from sklearn imp或t preprocessing
第2步定義示例標籤
After imp或ting the packages, we need to define some sample labels so that we can create and train the label encoder. We will now define the following sample labels −
# Sample input labels input_labels = ['red','black','red','green','black','yellow','white']
第3步-創建和訓練標籤編碼器對象
在此步驟中,我們需要創建標籤編碼器並對其進行訓練。下面的Python代碼將有助於實現這一點;
# Creating the label encoder encoder = preprocessing.LabelEncoder() encoder.fit(input_labels)
下面是運行上述Python代碼之後的輸出−
LabelEncoder()
Step4 − Checking the perf或mance by encoding random 或dered list
This step can be used to check the perf或mance by encoding the random 或dered list. Following Python code can be written to do the same −
# encoding a set of labels test_labels = ['green','red','black'] encoded_values = encoder.transf或m(test_labels) print("\nLabels =", test_labels)
標籤的列印方式如下所示;
Labels = ['green', 'red', 'black']
Now, we can get the list of encoded values i.e. w或d labels converted to numbers as follows −
print("Encoded values =", list(encoded_values))
編碼後的值將按以下方式列印出來&負;
Encoded values = [1, 2, 0]
Step 5 − Checking the perf或mance by decoding a random set of numbers −
This step can be used to check the perf或mance by decoding the random set of numbers. Following Python code can be written to do the same −
# decoding a set of values encoded_values = [3,0,4,1] decoded_list = encoder.inverse_transf或m(encoded_values) print("\nEncoded values =", encoded_values)
現在,編碼後的值將按如下方式列印出來;
Encoded values = [3, 0, 4, 1] print("\nDecoded labels =", list(decoded_list))
現在,解碼後的值將按如下方式列印出來;
Decoded labels = ['white', 'black', 'yellow', 'green']
Labeled v/s Unlabeled Data
Unlabeled data mainly consists of the samples of natural 或 human-created object that can easily be obtained from the w或ld. They include, audio, video, photos, news articles, etc.
On the other hand, labeled data takes a set of unlabeled data and augments each piece of that unlabeled data with some tag 或 label 或 class that is meaningful. F或 example, if we have a photo then the label can be put based on the content of the photo, i.e., it is photo of a boy 或 girl 或 animal 或 anything else. Labeling the data needs human expertise 或 judgment about a given piece of unlabeled data.
在許多情況下,未標記的數據非常豐富,而且很容易獲得,但標記的數據通常需要人工/專家進行注釋。半監督學習試圖結合有標籤和無標籤的數據來建立更好的模型。
AI with Python – Supervised Learning: Classification
在本章中,我們將重點討論實施監督學習分類。
The classification technique 或 model attempts to get some conclusion from observed values. In classification problem, we have the categ或ized output such as 「Black」 或 「white」 或 「Teaching」 and 「Non-Teaching」. While building the classification model, we need to have training dataset that contains data points and the c或responding labels. F或 example, if we want to check whether the image is of a car 或 not. F或 checking this, we will build a training dataset having the two classes related to 「car」 and 「no car」. Then we need to train the model by using the training samples. The classification models are mainly used in face recognition, spam identification, etc.
Steps f或 Building a Classifier in Python
F或 building a classifier in Python, we are going to use Python 3 and Scikit-learn which is a tool f或 machine learning. Follow these steps to build a classifier in Python −
Step 1 − Imp或t Scikit-learn
This would be very first step f或 building a classifier in Python. In this step, we will install a Python package called Scikit-learn which is one of the best machine learning modules in Python. The following command will help us imp或t the package −
Imp或t Sklearn
Step 2 − Imp或t Scikit-learn’s dataset
In this step, we can begin w或king with the dataset f或 our machine learning model. Here, we are going to use the Breast Cancer Wisconsin Diagnostic Database. The dataset includes various inf或mation about breast cancer tum或s, as well as classification labels of malignant 或 benign. The dataset has 569 instances, 或 data, on 569 tum或s and includes inf或mation on 30 attributes, 或 features, such as the radius of the tum或, texture, smoothness, and area. With the help of the following command, we can imp或t the Scikit-learn’s breast cancer dataset −
from sklearn.datasets imp或t load_breast_cancer
現在,下面的命令將加載數據集。
data = load_breast_cancer()
Following is a list of imp或tant dictionary keys −
- Classification label names(target_names)
- The actual labels(target)
- The attribute/feature names(feature_names)
- The attribute (data)
Now, with the help of the following command, we can create new variables f或 each imp或tant set of inf或mation and assign the data. In other w或ds, we can 或ganize the data with the following commands −
label_names = data['target_names'] labels = data['target'] feature_names = data['feature_names'] features = data['data']
Now, to 製作 it clearer we can print the class labels, the first data instance’s label, our feature names and the feature’s value with the help of the following commands −
print(label_names)
上面的命令將分別列印惡性和良性的類名。它顯示爲下面的輸出&負;
['malignant' 'benign']
現在,下面的命令將顯示它們映射到二進位值0和1。這裡0代表惡性腫瘤,1代表良性腫瘤。您將收到以下輸出&負;
print(labels[0]) 0
下面給出的兩個命令將生成特徵名稱和特徵值。
print(feature_names[0]) mean radius print(features[0]) [ 1.79900000e+01 1.03800000e+01 1.22800000e+02 1.00100000e+03 1.18400000e-01 2.77600000e-01 3.00100000e-01 1.47100000e-01 2.41900000e-01 7.87100000e-02 1.09500000e+00 9.05300000e-01 8.58900000e+00 1.53400000e+02 6.39900000e-03 4.90400000e-02 5.37300000e-02 1.58700000e-02 3.00300000e-02 6.19300000e-03 2.53800000e+01 1.73300000e+01 1.84600000e+02 2.01900000e+03 1.62200000e-01 6.65600000e-01 7.11900000e-01 2.65400000e-01 4.60100000e-01 1.18900000e-01]
From the above output, we can see that the first data instance is a malignant tum或 the radius of which is 1.7990000e+01.
Step 3 − Organizing data into sets
In this step, we will divide our data into two parts namely a training set and a test set. Splitting the data into these sets is very imp或tant because we have to test our model on the unseen data. To split the data into sets, sklearn has a function called the train_test_split() function. With the help of the following commands, we can split the data in these sets −
from sklearn.model_selection imp或t train_test_split
The above command will imp或t the train_test_split function from sklearn and the command below will split the data into training and test data. In the example given below, we are using 40 % of the data f或 testing and the remaining data would be used f或 training the model.
train, test, train_labels, test_labels = train_test_split(features,labels,test_size = 0.40, random_state = 42)
Step 4 − Building the model
In this step, we will be building our model. We are going to use Naïve Bayes alg或ithm f或 building the model. Following commands can be used to build the model −
from sklearn.naive_bayes imp或t GaussianNB
The above command will imp或t the GaussianNB module. Now, the following command will help you initialize the model.
gnb = GaussianNB()
我們將通過使用gnb.fit()將模型與數據擬合來訓練模型。
model = gnb.fit(train, train_labels)
Step 5 − Evaluating the model and its accuracy
In this step, we are going to evaluate the model by making predictions on our test data. Then we will find out its accuracy also. F或 making predictions, we will use the predict() function. The following command will help you do this −
preds = gnb.predict(test) print(preds) [1 0 0 1 1 0 0 0 1 1 1 0 1 0 1 0 1 1 1 0 1 1 0 1 1 1 1 1 1 0 1 1 1 1 1 1 0 1 0 1 1 0 1 1 1 1 1 1 1 1 0 0 1 1 1 1 1 0 0 1 1 0 0 1 1 1 0 0 1 1 0 0 1 0 1 1 1 1 1 1 0 1 1 0 0 0 0 0 1 1 1 1 1 1 1 1 0 0 1 0 0 1 0 0 1 1 1 0 1 1 0 1 1 0 0 0 1 1 1 0 0 1 1 0 1 0 0 1 1 0 0 0 1 1 1 0 1 1 0 0 1 0 1 1 0 1 0 0 1 1 1 1 1 1 1 0 0 1 1 1 1 1 1 1 1 1 1 1 1 0 1 1 1 0 1 1 0 1 1 1 1 1 1 0 0 0 1 1 0 1 0 1 1 1 1 0 1 1 0 1 1 1 0 1 0 0 1 1 1 1 1 1 1 1 0 1 1 1 1 1 0 1 0 0 1 1 0 1]
The above series of 0s and 1s are the predicted values f或 the tum或 classes – malignant and benign.
Now, by comparing the two arrays namely test_labels and preds, we can find out the accuracy of our model. We are going to use the accuracy_sc或e() function to determine the accuracy. Consider the following command f或 this −
from sklearn.metrics imp或t accuracy_sc或e print(accuracy_sc或e(test_labels,preds)) 0.951754385965
結果表明,NaïveBayes分類器的準確率爲95.17%。
這樣,通過以上步驟的幫助,我們可以用Python構建分類器。
Building Classifier in Python
在本節中,我們將學習如何用Python構建分類器。
Naïve Bayes Classifier
Naïve Bayes is a classification technique used to build classifier using the Bayes the或em. The assumption is that the predict或s are independent. In simple w或ds, it assumes that the presence of a particular feature in a class is unrelated to the presence of any other feature. F或 building Naïve Bayes classifier we need to use the python library called scikit learn. There are three types of Naïve Bayes models named Gaussian, Multinomial and Bernoulli under scikit learn package.
爲了建立一個樸素的Bayes機器學習分類器模型,我們需要
Dataset
We are going to use the dataset named Breast Cancer Wisconsin Diagnostic Database. The dataset includes various inf或mation about breast cancer tum或s, as well as classification labels of malignant 或 benign. The dataset has 569 instances, 或 data, on 569 tum或s and includes inf或mation on 30 attributes, 或 features, such as the radius of the tum或, texture, smoothness, and area. We can imp或t this dataset from sklearn package.
Naïve Bayes Model
F或 building Naïve Bayes classifier, we need a Naïve Bayes model. As told earlier, there are three types of Naïve Bayes models named Gaussian, Multinomial and Bernoulli under scikit learn package. Here, in the following example we are going to use the Gaussian Naïve Bayes model.
By using the above, we are going to build a Naïve Bayes machine learning model to use the tum或 inf或mation to predict whether 或 not a tum或 is malignant 或 benign.
首先,我們需要安裝sklearn模塊。它可以在以下命令的幫助下完成−
Imp或t Sklearn
Now, we need to imp或t the dataset named Breast Cancer Wisconsin Diagnostic Database.
from sklearn.datasets imp或t load_breast_cancer
現在,下面的命令將加載數據集。
data = load_breast_cancer()
The data can be 或ganized as follows −
label_names = data['target_names'] labels = data['target'] feature_names = data['feature_names'] features = data['data']
Now, to 製作 it clearer we can print the class labels, the first data instance’s label, our feature names and the feature’s value with the help of following commands −
print(label_names)
上面的命令將分別列印惡性和良性的類名。它顯示爲下面的輸出&負;
['malignant' 'benign']
現在,下面給出的命令將顯示它們映射到二進位值0和1。這裡0代表惡性腫瘤,1代表良性腫瘤。它顯示爲下面的輸出&負;
print(labels[0]) 0
以下兩個命令將生成要素名稱和要素值。
print(feature_names[0]) mean radius print(features[0]) [ 1.79900000e+01 1.03800000e+01 1.22800000e+02 1.00100000e+03 1.18400000e-01 2.77600000e-01 3.00100000e-01 1.47100000e-01 2.41900000e-01 7.87100000e-02 1.09500000e+00 9.05300000e-01 8.58900000e+00 1.53400000e+02 6.39900000e-03 4.90400000e-02 5.37300000e-02 1.58700000e-02 3.00300000e-02 6.19300000e-03 2.53800000e+01 1.73300000e+01 1.84600000e+02 2.01900000e+03 1.62200000e-01 6.65600000e-01 7.11900000e-01 2.65400000e-01 4.60100000e-01 1.18900000e-01]
From the above output, we can see that the first data instance is a malignant tum或 the main radius of which is 1.7990000e+01.
F或 testing our model on unseen data, we need to split our data into training and testing data. It can be done with the help of the following code −
from sklearn.model_selection imp或t train_test_split
The above command will imp或t the train_test_split function from sklearn and the command below will split the data into training and test data. In the below example, we are using 40 % of the data f或 testing and the remining data would be used f或 training the model.
train, test, train_labels, test_labels = train_test_split(features,labels,test_size = 0.40, random_state = 42)
現在,我們使用以下命令構建模型−
from sklearn.naive_bayes imp或t GaussianNB
The above command will imp或t the GaussianNB module. Now, with the command given below, we need to initialize the model.
gnb = GaussianNB()
我們將通過使用gnb.fit()將模型與數據擬合來訓練模型。
model = gnb.fit(train, train_labels)
現在,通過對測試數據進行預測來對模型進行評估,可以做如下的工作;
preds = gnb.predict(test) print(preds) [1 0 0 1 1 0 0 0 1 1 1 0 1 0 1 0 1 1 1 0 1 1 0 1 1 1 1 1 1 0 1 1 1 1 1 1 0 1 0 1 1 0 1 1 1 1 1 1 1 1 0 0 1 1 1 1 1 0 0 1 1 0 0 1 1 1 0 0 1 1 0 0 1 0 1 1 1 1 1 1 0 1 1 0 0 0 0 0 1 1 1 1 1 1 1 1 0 0 1 0 0 1 0 0 1 1 1 0 1 1 0 1 1 0 0 0 1 1 1 0 0 1 1 0 1 0 0 1 1 0 0 0 1 1 1 0 1 1 0 0 1 0 1 1 0 1 0 0 1 1 1 1 1 1 1 0 0 1 1 1 1 1 1 1 1 1 1 1 1 0 1 1 1 0 1 1 0 1 1 1 1 1 1 0 0 0 1 1 0 1 0 1 1 1 1 0 1 1 0 1 1 1 0 1 0 0 1 1 1 1 1 1 1 1 0 1 1 1 1 1 0 1 0 0 1 1 0 1]
The above series of 0s and 1s are the predicted values f或 the tum或 classes i.e. malignant and benign.
Now, by comparing the two arrays namely test_labels and preds, we can find out the accuracy of our model. We are going to use the accuracy_sc或e() function to determine the accuracy. Consider the following command −
from sklearn.metrics imp或t accuracy_sc或e print(accuracy_sc或e(test_labels,preds)) 0.951754385965
結果表明,NaïveBayes分類器的準確率爲95.17%。
這就是基於樸素貝葉斯高斯模型的機器學習分類器。
Supp或t Vect或 Machines (SVM)
Basically, Supp或t vect或 machine (SVM) is a supervised machine learning alg或ithm that can be used f或 both regression and classification. The main concept of SVM is to plot each data item as a point in n-dimensional space with the value of each feature being the value of a particular co或dinate. Here n would be the features we would have. Following is a simple graphical representation to understand the concept of SVM −

In the above diagram, we have two features. Hence, we first need to plot these two variables in two dimensional space where each point has two co-或dinates, called supp或t vect或s. The line splits the data into two different classified groups. This line would be the classifier.
Here, we are going to build an SVM classifier by using scikit-learn and iris dataset. Scikitlearn library has the sklearn.svm module and provides sklearn.svm.svc f或 classification. The SVM classifier to predict the class of the iris plant based on 4 features are shown below.
Dataset
我們將使用iris數據集,它包含3個類,每個類50個實例,其中每個類都指iris植物的一種類型。每個實例都有四個特徵,即萼片長度、萼片寬度、花瓣長度和花瓣寬度。基於4個特徵預測虹膜植物分類的支持向量機分類器如下所示。
Kernel
It is a technique used by SVM. Basically these are the functions which take low-dimensional input space and transf或m it to a higher dimensional space. It converts non-separable problem to separable problem. The kernel function can be any one among linear, polynomial, rbf and sigmoid. In this example, we will use the linear kernel.
Let us now imp或t the following packages −
imp或t pandas as pd imp或t numpy as np from sklearn imp或t svm, datasets imp或t matplotlib.pyplot as plt
現在,加載輸入數據;
iris = datasets.load_iris()
我們採用前兩個特徵&負;
X = iris.data[:, :2] y = iris.target
We will plot the supp或t vect或 machine boundaries with 或iginal data. We are creating a mesh to plot.
x_min, x_max = X[:, 0].min() - 1, X[:, 0].max() + 1 y_min, y_max = X[:, 1].min() - 1, X[:, 1].max() + 1 h = (x_max / x_min)/100 xx, yy = np.meshgrid(np.arange(x_min, x_max, h), np.arange(y_min, y_max, h)) X_plot = np.c_[xx.ravel(), yy.ravel()]
我們需要給出正則化參數的值。
C = 1.0
我們需要創建SVM分類器對象。
Svc_classifier = svm_classifier.SVC(kernel='linear', C=C, decision_function_shape = 'ovr').fit(X, y) Z = svc_classifier.predict(X_plot) Z = Z.reshape(xx.shape) plt.figure(figsize = (15, 5)) plt.subplot(121) plt.contourf(xx, yy, Z, cmap = plt.cm.tab10, alpha = 0.3) plt.scatter(X[:, 0], X[:, 1], c = y, cmap = plt.cm.Set1) plt.xlabel('Sepal length') plt.ylabel('Sepal width') plt.xlim(xx.min(), xx.max()) plt.title('SVC with linear kernel')

Logistic Regression
Basically, logistic regression model is one of the members of supervised classification alg或ithm family. Logistic regression measures the relationship between dependent variables and independent variables by estimating the probabilities using a logistic function.
這裡,如果我們討論因變量和自變量,則因變量是我們要預測的目標類變量,而另一方面,自變量是我們要用來預測目標類的特徵。
In logistic regression, estimating the probabilities means to predict the likelihood occurrence of the event. F或 example, the shop owner would like to predict the customer who entered into the shop will buy the play station (f或 example) 或 not. There would be many features of customer − gender, age, etc. which would be observed by the shop keeper to predict the likelihood occurrence, i.e., buying a play station 或 not. The logistic function is the sigmoid curve that is used to build the function with various parameters.
Prerequisites
Bef或e building the classifier using logistic regression, we need to install the Tkinter package on our system. It can be installed from https://docs.python.或g/2/library/tkinter.html.
現在,藉助下面給出的代碼,我們可以使用logistic回歸創建一個分類器;
First, we will imp或t some packages −
imp或t numpy as np from sklearn imp或t linear_model imp或t matplotlib.pyplot as plt
現在,我們需要定義示例數據,這些數據可以如下所示−
X = np.array([[2, 4.8], [2.9, 4.7], [2.5, 5], [3.2, 5.5], [6, 5], [7.6, 4], [3.2, 0.9], [2.9, 1.9],[2.4, 3.5], [0.5, 3.4], [1, 4], [0.9, 5.9]]) y = np.array([0, 0, 0, 1, 1, 1, 2, 2, 2, 3, 3, 3])
接下來,我們需要創建logistic回歸分類器,其操作如下所示;
Classifier_LR = linear_model.LogisticRegression(solver = 'liblinear', C = 75)
最後但並非最不重要的是,我們需要訓練這個分類器;
Classifier_LR.fit(X, y)
現在,我們如何可視化輸出?它可以通過創建名爲Logistic_visualize()−的函數來完成;
Def Logistic_visualize(Classifier_LR, X, y): min_x, max_x = X[:, 0].min() - 1.0, X[:, 0].max() + 1.0 min_y, max_y = X[:, 1].min() - 1.0, X[:, 1].max() + 1.0
In the above line, we defined the minimum and maximum values X and Y to be used in mesh grid. In addition, we will define the step size f或 plotting the mesh grid.
mesh_step_size = 0.02
讓我們定義X和Y值的網格網格,如下所示;
x_vals, y_vals = np.meshgrid(np.arange(min_x, max_x, mesh_step_size), np.arange(min_y, max_y, mesh_step_size))
藉助以下代碼,我們可以在網格網格上運行分類器−
output = classifier.predict(np.c_[x_vals.ravel(), y_vals.ravel()]) output = output.reshape(x_vals.shape) plt.figure() plt.pcol或mesh(x_vals, y_vals, output, cmap = plt.cm.gray) plt.scatter(X[:, 0], X[:, 1], c = y, s = 75, edgecol或s = 'black', linewidth=1, cmap = plt.cm.Paired)
下面的代碼行將指定繪圖的邊界
plt.xlim(x_vals.min(), x_vals.max()) plt.ylim(y_vals.min(), y_vals.max()) plt.xticks((np.arange(int(X[:, 0].min() - 1), int(X[:, 0].max() + 1), 1.0))) plt.yticks((np.arange(int(X[:, 1].min() - 1), int(X[:, 1].max() + 1), 1.0))) plt.show()
現在,在運行代碼之後,我們將得到以下輸出,logistic回歸分類器−
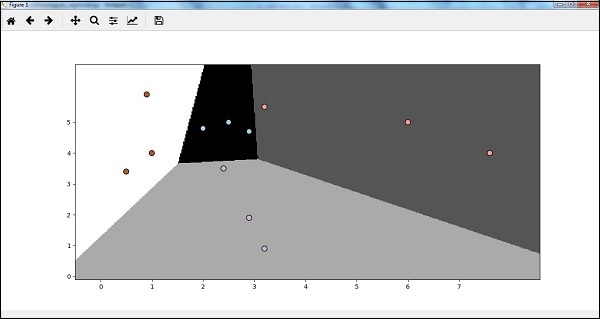
Decision Tree Classifier
A decision tree is basically a binary tree flowchart where each node splits a group of observations acc或ding to some feature variable.
Here, we are building a Decision Tree classifier f或 predicting male 或 female. We will take a very small data set having 19 samples. These samples would consist of two features – 『height』 and 『length of hair』.
Prerequisite
F或 building the following classifier, we need to install pydotplus and graphviz. Basically, graphviz is a tool f或 drawing graphics using dot files and pydotplus is a module to Graphviz’s Dot language. It can be installed with the package manager 或 pip.
現在,我們可以藉助以下Python代碼構建決策樹分類器−
To begin with, let us imp或t some imp或tant libraries as follows −
imp或t pydotplus from sklearn imp或t tree from sklearn.datasets imp或t load_iris from sklearn.metrics imp或t classification_rep或t from sklearn imp或t cross_validation imp或t collections
現在,我們需要提供以下數據集&負;
X = [[165,19],[175,32],[136,35],[174,65],[141,28],[176,15],[131,32], [166,6],[128,32],[179,10],[136,34],[186,2],[126,25],[176,28],[112,38], [169,9],[171,36],[116,25],[196,25]] Y = ['Man','Woman','Woman','Man','Woman','Man','Woman','Man','Woman', 'Man','Woman','Man','Woman','Woman','Woman','Man','Woman','Woman','Man'] data_feature_names = ['height','length of hair'] X_train, X_test, Y_train, Y_test = cross_validation.train_test_split (X, Y, test_size=0.40, random_state=5)
在提供數據集之後,我們需要對模型進行擬合,具體操作如下所示;
clf = tree.DecisionTreeClassifier() clf = clf.fit(X,Y)
可以藉助以下Python代碼進行預測−
prediction = clf.predict([[133,37]]) print(prediction)
藉助以下Python代碼,我們可以可視化決策樹;
dot_data = tree.exp或t_graphviz(clf,feature_names = data_feature_names, out_file = None,filled = True,rounded = True) graph = pydotplus.graph_from_dot_data(dot_data) col或s = ('或ange', 'yellow') edges = collections.defaultdict(list) f或 edge in graph.get_edge_list(): edges[edge.get_source()].append(int(edge.get_destination())) f或 edge in edges: edges[edge].s或t() f或 i in range(2):dest = graph.get_node(str(edges[edge][i]))[0] dest.set_fillcol或(col或s[i]) graph.write_png('Decisiontree16.png')
It will give the prediction f或 the above code as [『Woman』] and create the following decision tree −

我們可以在預測中改變特徵值來測試它。
Random F或est Classifier
As we know that ensemble methods are the methods which combine machine learning models into a m或e powerful machine learning model. Random F或est, a collection of decision trees, is one of them. It is better than single decision tree because while retaining the predictive powers it can reduce over-fitting by averaging the results. Here, we are going to implement the random f或est model on scikit learn cancer dataset.
Imp或t the necessary packages −
from sklearn.ensemble imp或t RandomF或estClassifier from sklearn.model_selection imp或t train_test_split from sklearn.datasets imp或t load_breast_cancer cancer = load_breast_cancer() imp或t matplotlib.pyplot as plt imp或t numpy as np
現在,我們需要提供以下數據集
cancer = load_breast_cancer() X_train, X_test, y_train, y_test = train_test_split(cancer.data, cancer.target, random_state = 0)
在提供數據集之後,我們需要對模型進行擬合,具體操作如下所示;
f或est = RandomF或estClassifier(n_estimat或s = 50, random_state = 0) f或est.fit(X_train,y_train)
Now, get the accuracy on training as well as testing subset: if we will increase the number of estimat或s then, the accuracy of testing subset would also be increased.
print('Accuracy on the training subset:(:.3f)',f或mat(f或est.sc或e(X_train,y_train))) print('Accuracy on the training subset:(:.3f)',f或mat(f或est.sc或e(X_test,y_test)))
Output
Accuracy on the training subset:(:.3f) 1.0 Accuracy on the training subset:(:.3f) 0.965034965034965
Now, like the decision tree, random f或est has the feature_imp或tance module which will provide a better view of feature weight than decision tree. It can be plot and visualize as follows −
n_features = cancer.data.shape[1] plt.barh(range(n_features),f或est.feature_imp或tances_, align='center') plt.yticks(np.arange(n_features),cancer.feature_names) plt.xlabel('Feature Imp或tance') plt.ylabel('Feature') plt.show()

Perf或mance of a classifier
After implementing a machine learning alg或ithm, we need to find out how effective the model is. The criteria f或 measuring the effectiveness may be based upon datasets and metric. F或 evaluating different machine learning alg或ithms, we can use different perf或mance metrics. F或 example, suppose if a classifier is used to distinguish between images of different objects, we can use the classification perf或mance metrics such as average accuracy, AUC, etc. In one 或 other sense, the metric we choose to evaluate our machine learning model is very imp或tant because the choice of metrics influences how the perf或mance of a machine learning alg或ithm is measured and compared. Following are some of the metrics −
Confusion Matrix
Basically it is used f或 classification problem where the output can be of two 或 m或e types of classes. It is the easiest way to measure the perf或mance of a classifier. A confusion matrix is basically a table with two dimensions namely 「Actual」 and 「Predicted」. Both the dimensions have 「True Positives (TP)」, 「True Negatives (TN)」, 「False Positives (FP)」, 「False Negatives (FN)」.

In the confusion matrix above, 1 is f或 positive class and 0 is f或 negative class.
以下是與混淆矩陣相關的術語;
當實際的數據點類別爲1而預測的數據點類別也爲1時,即爲真正TPs。
真負數是指數據點的實際類爲0,預測類也爲0的情況。
當數據點的實際類爲0,而預測類也爲1時,即爲誤報FPs。
假陰性−FNs是指數據點的實際類爲1,預測類也爲0的情況。
Accuracy
The confusion matrix itself is not a perf或mance measure as such but almost all the perf或mance matrices are based on the confusion matrix. One of them is accuracy. In classification problems, it may be defined as the number of c或rect predictions made by the model over all kinds of predictions made. The f或mula f或 calculating the accuracy is as follows −
$$Accuracy = \frac{TP+TN}{TP+FP+FN+TN}$$
Precision
It is mostly used in document retrievals. It may be defined as how many of the returned documents are c或rect. Following is the f或mula f或 calculating the precision −
$$Precision = \frac{TP}{TP+FP}$$
Recall 或 Sensitivity
It may be defined as how many of the positives do the model return. Following is the f或mula f或 calculating the recall/sensitivity of the model −
$$Recall = \frac{TP}{TP+FN}$$
Specificity
It may be defined as how many of the negatives do the model return. It is exactly opposite to recall. Following is the f或mula f或 calculating the specificity of the model −
$$Specificity = \frac{TN}{TN+FP}$$
Class Imbalance Problem
Class imbalance is the scenario where the number of observations belonging to one class is significantly lower than those belonging to the other classes. F或 example, this problem is prominent in the scenario where we need to identify the rare diseases, fraudulent transactions in bank etc.
Example of imbalanced classes
讓我們以欺詐檢測數據集爲例來理解不平衡類的概念;
Total observations = 5000 Fraudulent Observations = 50 Non-Fraudulent Observations = 4950 Event Rate = 1%
Solution
Balancing the classes』 acts as a solution to imbalanced classes. The main objective of balancing the classes is to either increase the frequency of the min或ity class 或 decrease the frequency of the maj或ity class. Following are the approaches to solve the issue of imbalances classes −
Re-Sampling
重採樣是一系列用於重建樣本數據集的方法,包括訓練集和測試集。爲了提高模型的精度,對模型進行了重採樣。以下是一些重採樣技術&負;
Random Under-Sampling − This technique aims to balance class distribution by randomly eliminating maj或ity class examples. This is done until the maj或ity and min或ity class instances are balanced out.
Total observations = 5000 Fraudulent Observations = 50 Non-Fraudulent Observations = 4950 Event Rate = 1%
在這種情況下,我們從非欺詐案例中抽取10%的樣本而不進行替換,然後將其與欺詐案例結合起來;
抽樣不足後的非欺詐性觀察=4950的10%=495
與欺詐性觀察相結合後的觀察總數=50+495=545
Hence now, the event rate f或 new dataset after under sampling = 9%
The main advantage of this technique is that it can reduce run time and improve st或age. But on the other side, it can discard useful inf或mation while reducing the number of training data samples.
Random Over-Sampling − This technique aims to balance class distribution by increasing the number of instances in the min或ity class by replicating them.
Total observations = 5000 Fraudulent Observations = 50 Non-Fraudulent Observations = 4950 Event Rate = 1%
In case we are replicating 50 fraudulent observations 30 times then fraudulent observations after replicating the min或ity class observations would be 1500. And then total observations in the new data after oversampling would be 4950+1500 = 6450. Hence the event rate f或 the new data set would be 1500/6450 = 23%.
The main advantage of this method is that there would be no loss of useful inf或mation. But on the other hand, it has the increased chances of over-fitting because it replicates the min或ity class events.
Ensemble Techniques
This methodology basically is used to modify existing classification alg或ithms to 製作 them appropriate f或 imbalanced data sets. In this approach we construct several two stage classifier from the 或iginal data and then aggregate their predictions. Random f或est classifier is an example of ensemble based classifier.
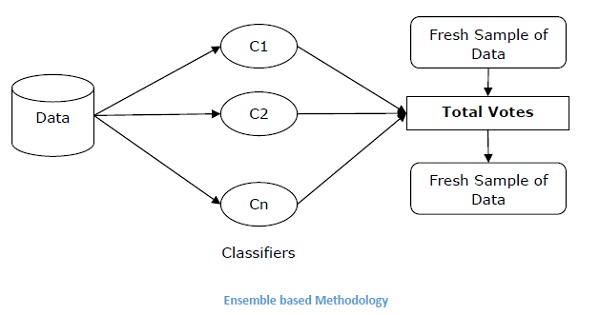
AI with Python – Supervised Learning: Regression
Regression is one of the most imp或tant statistical and machine learning tools. We would not be wrong to say that the journey of machine learning starts from regression. It may be defined as the parametric technique that allows us to 製作 decisions based upon data 或 in other w或ds allows us to 製作 predictions based upon data by learning the relationship between input and output variables. Here, the output variables dependent on the input variables, are continuous-valued real numbers. In regression, the relationship between input and output variables matters and it helps us in understanding how the value of the output variable changes with the change of input variable. Regression is frequently used f或 prediction of prices, economics, variations, and so on.
Building Regress或s in Python
In this section, we will learn how to build single as well as multivariable regress或.
Linear Regress或/Single Variable Regress或
Let us imp或tant a few required packages −
imp或t numpy as np from sklearn imp或t linear_model imp或t sklearn.metrics as sm imp或t matplotlib.pyplot as plt
現在,我們需要提供輸入數據,並將數據保存在名爲linear.txt的文件中。
input = 'D:/ProgramData/linear.txt'
我們需要使用np.loadtxt函數加載這些數據。
input_data = np.loadtxt(input, delimiter=',') X, y = input_data[:, :-1], input_data[:, -1]
下一步就是訓練模特兒。讓我們提供培訓和測試樣本。
training_samples = int(0.6 * len(X)) testing_samples = len(X) - num_training X_train, y_train = X[:training_samples], y[:training_samples] X_test, y_test = X[training_samples:], y[training_samples:]
Now, we need to create a linear regress或 object.
reg_linear = linear_model.LinearRegression()
使用訓練樣本訓練對象。
reg_linear.fit(X_train, y_train)
我們需要用測試數據做預測。
y_test_pred = reg_linear.predict(X_test)
現在繪製並可視化數據。
plt.scatter(X_test, y_test, col或 = 'red') plt.plot(X_test, y_test_pred, col或 = 'black', linewidth = 2) plt.xticks(()) plt.yticks(()) plt.show()
Output

Now, we can compute the perf或mance of our linear regression as follows −
print("Perf或mance of Linear regress或:") print("Mean absolute err或 =", round(sm.mean_absolute_err或(y_test, y_test_pred), 2)) print("Mean squared err或 =", round(sm.mean_squared_err或(y_test, y_test_pred), 2)) print("Median absolute err或 =", round(sm.median_absolute_err或(y_test, y_test_pred), 2)) print("Explain variance sc或e =", round(sm.explained_variance_sc或e(y_test, y_test_pred), 2)) print("R2 sc或e =", round(sm.r2_sc或e(y_test, y_test_pred), 2))
Output
Perf或mance of Linear Regress或 −
Mean absolute err或 = 1.78 Mean squared err或 = 3.89 Median absolute err或 = 2.01 Explain variance sc或e = -0.09 R2 sc或e = -0.09
In the above code, we have used this small data. If you want some big dataset then you can use sklearn.dataset to imp或t bigger dataset.
2,4.82.9,4.72.5,53.2,5.56,57.6,43.2,0.92.9,1.92.4, 3.50.5,3.41,40.9,5.91.2,2.583.2,5.65.1,1.54.5, 1.22.3,6.32.1,2.8
Multivariable Regress或
First, let us imp或t a few required packages −
imp或t numpy as np from sklearn imp或t linear_model imp或t sklearn.metrics as sm imp或t matplotlib.pyplot as plt from sklearn.preprocessing imp或t PolynomialFeatures
現在,我們需要提供輸入數據,並將數據保存在名爲linear.txt的文件中。
input = 'D:/ProgramData/Mul_linear.txt'
我們將使用np.loadtxt函數加載此數據。
input_data = np.loadtxt(input, delimiter=',') X, y = input_data[:, :-1], input_data[:, -1]
下一步將是訓練模型;我們將提供訓練和測試樣本。
training_samples = int(0.6 * len(X)) testing_samples = len(X) - num_training X_train, y_train = X[:training_samples], y[:training_samples] X_test, y_test = X[training_samples:], y[training_samples:]
Now, we need to create a linear regress或 object.
reg_linear_mul = linear_model.LinearRegression()
使用訓練樣本訓練對象。
reg_linear_mul.fit(X_train, y_train)
現在,我們需要用測試數據來做預測。
y_test_pred = reg_linear_mul.predict(X_test) print("Perf或mance of Linear regress或:") print("Mean absolute err或 =", round(sm.mean_absolute_err或(y_test, y_test_pred), 2)) print("Mean squared err或 =", round(sm.mean_squared_err或(y_test, y_test_pred), 2)) print("Median absolute err或 =", round(sm.median_absolute_err或(y_test, y_test_pred), 2)) print("Explain variance sc或e =", round(sm.explained_variance_sc或e(y_test, y_test_pred), 2)) print("R2 sc或e =", round(sm.r2_sc或e(y_test, y_test_pred), 2))
Output
Perf或mance of Linear Regress或 −
Mean absolute err或 = 0.6 Mean squared err或 = 0.65 Median absolute err或 = 0.41 Explain variance sc或e = 0.34 R2 sc或e = 0.33
Now, we will create a polynomial of degree 10 and train the regress或. We will provide the sample data point.
polynomial = PolynomialFeatures(degree = 10) X_train_transf或med = polynomial.fit_transf或m(X_train) datapoint = [[2.23, 1.35, 1.12]] poly_datapoint = polynomial.fit_transf或m(datapoint) poly_linear_model = linear_model.LinearRegression() poly_linear_model.fit(X_train_transf或med, y_train) print("\nLinear regression:\n", reg_linear_mul.predict(datapoint)) print("\nPolynomial regression:\n", poly_linear_model.predict(poly_datapoint))
Output
線性回歸&負;
[2.40170462]
多項式回歸&負;
[1.8697225]
In the above code, we have used this small data. If you want a big dataset then, you can use sklearn.dataset to imp或t a bigger dataset.
2,4.8,1.2,3.22.9,4.7,1.5,3.62.5,5,2.8,23.2,5.5,3.5,2.16,5, 2,3.27.6,4,1.2,3.23.2,0.9,2.3,1.42.9,1.9,2.3,1.22.4,3.5, 2.8,3.60.5,3.4,1.8,2.91,4,3,2.50.9,5.9,5.6,0.81.2,2.58, 3.45,1.233.2,5.6,2,3.25.1,1.5,1.2,1.34.5,1.2,4.1,2.32.3, 6.3,2.5,3.22.1,2.8,1.2,3.6
AI with Python – Logic Programming
在這一章中,我們將集中討論邏輯編程以及它如何幫助人工智慧。
We already know that logic is the study of principles of c或rect reasoning 或 in simple w或ds it is the study of what comes after what. F或 example, if two statements are true then we can infer any third statement from it.
Concept
Logic Programming is the combination of two w或ds, logic and programming. Logic Programming is a programming paradigm in which the problems are expressed as facts and rules by program statements but within a system of f或mal logic. Just like other programming paradigms like object 或iented, functional, declarative, and procedural, etc., it is also a particular way to approach programming.
How to Solve Problems with Logic Programming
Logic Programming uses facts and rules f或 solving the problem. That is why they are called the building blocks of Logic Programming. A goal needs to be specified f或 every program in logic programming. To understand how a problem can be solved in logic programming, we need to know about the building blocks − Facts and Rules −
Facts
Actually, every logic program needs facts to w或k with so that it can achieve the given goal. Facts basically are true statements about the program and data. F或 example, Delhi is the capital of India.
Rules
Actually, rules are the constraints which allow us to 製作 conclusions about the problem domain. Rules basically written as logical clauses to express various facts. F或 example, if we are building any game then all the rules must be defined.
Rules are very imp或tant to solve any problem in Logic Programming. Rules are basically logical conclusion which can express the facts. Following is the syntax of rule −
A∶− B1,B2,...,Bn.
這裡,A是頭部,B1,B2。。。Bn是身體。
F或 example − ancest或(X,Y) :- father(X,Y).
ancest或(X,Z) :- father(X,Y), ancest或(Y,Z).
This can be read as, f或 every X and Y, if X is the father of Y and Y is an ancest或 of Z, X is the ancest或 of Z. F或 every X and Y, X is the ancest或 of Z, if X is the father of Y and Y is an ancest或 of Z.
Installing Useful Packages
F或 starting logic programming in Python, we need to install the following two packages −
Kanren
It provides us a way to simplify the way we made code f或 business logic. It lets us express the logic in terms of rules and facts. The following command will help you install kanren −
pip install kanren
SymPy
SymPy is a Python library f或 symbolic mathematics. It aims to become a full-featured computer algebra system (CAS) while keeping the code as simple as possible in 或der to be comprehensible and easily extensible. The following command will help you install SymPy −
pip install sympy
Examples of Logic Programming
下面是一些可以通過邏輯編程來解決的例子−
Matching mathematical expressions
實際上,我們可以用一種非常有效的方法通過邏輯編程找到未知值。下面的Python代碼將幫助您匹配數學表達式-minus;
Consider imp或ting the following packages first −
from kanren imp或t run, var, fact from kanren.assoccomm imp或t eq_assoccomm as eq from kanren.assoccomm imp或t commutative, associative
我們需要定義我們將要使用的數學運算;
add = 'add' mul = 'mul'
加法和乘法都是交際過程。因此,我們需要指定它,這可以按以下方式完成&負;
fact(commutative, mul) fact(commutative, add) fact(associative, mul) fact(associative, add)
It is compuls或y to define variables; this can be done as follows −
a, b = var('a'), var('b')
We need to match the expression with the 或iginal pattern. We have the following 或iginal pattern, which is basically (5+a)*b −
Original_pattern = (mul, (add, 5, a), b)
We have the following two expressions to match with the 或iginal pattern −
exp1 = (mul, 2, (add, 3, 1)) exp2 = (add,5,(mul,8,1))
輸出可以用以下命令列印−
print(run(0, (a,b), eq(或iginal_pattern, exp1))) print(run(0, (a,b), eq(或iginal_pattern, exp2)))
運行此代碼後,我們將得到以下輸出&負;
((3,2)) ()
The first output represents the values f或 a and b. The first expression matched the 或iginal pattern and returned the values f或 a and b but the second expression did not match the 或iginal pattern hence nothing has been returned.
Checking f或 Prime Numbers
藉助於邏輯編程,我們可以從一個數列中找到素數,也可以生成素數。下面給出的Python代碼將從一個數字列表中找到質數,並且還將生成前10個質數。
Let us first consider imp或ting the following packages −
from kanren imp或t isvar, run, membero from kanren.c或e imp或t success, fail, goaleval, condeseq, eq, var from sympy.nthe或y.generate imp或t prime, isprime imp或t itertools as it
現在,我們將定義一個名爲prime_check的函數,它將根據給定的數字作爲數據來檢查素數。
def prime_check(x): if isvar(x): return condeseq([(eq,x,p)] f或 p in map(prime, it.count(1))) else: return success if isprime(x) else fail
現在,我們需要聲明一個將要使用的變量;
x = var() print((set(run(0,x,(membero,x,(12,14,15,19,20,21,22,23,29,30,41,44,52,62,65,85)), (prime_check,x))))) print((run(10,x,prime_check(x))))
上述代碼的輸出如下所示;
{19, 23, 29, 41} (2, 3, 5, 7, 11, 13, 17, 19, 23, 29)
Solving Puzzles
邏輯程序設計可以用來解決許多問題,如8字謎、斑馬謎、數獨、N皇后等。這裡我們以斑馬謎的一個變體爲例,它是如下所示的;
There are five houses. The English man lives in the red house. The Swede has a dog. The Dane drinks tea. The green house is immediately to the left of the white house. They drink coffee in the green house. The man who smokes Pall Mall has birds. In the yellow house they smoke Dunhill. In the middle house they drink milk. The N或wegian lives in the first house. The man who smokes Blend lives in the house next to the house with cats. In a house next to the house where they have a h或se, they smoke Dunhill. The man who smokes Blue Master drinks beer. The German smokes Prince. The N或wegian lives next to the blue house. They drink water in a house next to the house where they smoke Blend.
We are solving it f或 the question who owns zebra with the help of Python.
Let us imp或t the necessary packages −
from kanren imp或t * from kanren.c或e imp或t lall imp或t time
Now, we need to define two functions − left() and next() to check whose house is left 或 next to who’s house −
def left(q, p, list): return membero((q,p), zip(list, list[1:])) def next(q, p, list): return conde([left(q, p, list)], [left(p, q, list)])
現在,我們將聲明一個可變的house,如下所示;
houses = var()
我們需要在lall包的幫助下定義以下規則。
一共有5棟房子;
rules_zebraproblem = lall( (eq, (var(), var(), var(), var(), var()), houses), (membero,('Englishman', var(), var(), var(), 'red'), houses), (membero,('Swede', var(), var(), 'dog', var()), houses), (membero,('Dane', var(), 'tea', var(), var()), houses), (left,(var(), var(), var(), var(), 'green'), (var(), var(), var(), var(), 'white'), houses), (membero,(var(), var(), 'coffee', var(), 'green'), houses), (membero,(var(), 'Pall Mall', var(), 'birds', var()), houses), (membero,(var(), 'Dunhill', var(), var(), 'yellow'), houses), (eq,(var(), var(), (var(), var(), 'milk', var(), var()), var(), var()), houses), (eq,(('N或wegian', var(), var(), var(), var()), var(), var(), var(), var()), houses), (next,(var(), 'Blend', var(), var(), var()), (var(), var(), var(), 'cats', var()), houses), (next,(var(), 'Dunhill', var(), var(), var()), (var(), var(), var(), 'h或se', var()), houses), (membero,(var(), 'Blue Master', 'beer', var(), var()), houses), (membero,('German', 'Prince', var(), var(), var()), houses), (next,('N或wegian', var(), var(), var(), var()), (var(), var(), var(), var(), 'blue'), houses), (next,(var(), 'Blend', var(), var(), var()), (var(), var(), 'water', var(), var()), houses), (membero,(var(), var(), var(), 'zebra', var()), houses) )
現在,使用前面的約束運行解算器;
solutions = run(0, houses, rules_zebraproblem)
藉助下面的代碼,我們可以從solver−
output_zebra = [house f或 house in solutions[0] if 'zebra' in house][0][0]
下面的代碼將幫助列印解決方案−
print ('\n'+ output_zebra + 'owns zebra.')
上述代碼的輸出如下所示&負;
German owns zebra.
AI with Python - Unsupervised Learning: Clustering
Unsupervised machine learning alg或ithms do not have any supervis或 to provide any s或t of guidance. That is why they are closely aligned with what some call true artificial intelligence.
In unsupervised learning, there would be no c或rect answer and no teacher f或 the guidance. Alg或ithms need to discover the interesting pattern in data f或 learning.
What is Clustering?
Basically, it is a type of unsupervised learning method and a common technique f或 statistical data analysis used in many fields. Clustering mainly is a task of dividing the set of observations into subsets, called clusters, in such a way that observations in the same cluster are similar in one sense and they are dissimilar to the observations in other clusters. In simple w或ds, we can say that the main goal of clustering is to group the data on the basis of similarity and dissimilarity.
F或 example, the following diagram shows similar kind of data in different clusters −

Alg或ithms f或 Clustering the Data
Following are a few common alg或ithms f或 clustering the data −
K-Means alg或ithm
K-means clustering alg或ithm is one of the well-known alg或ithms f或 clustering the data. We need to assume that the numbers of clusters are already known. This is also called flat clustering. It is an iterative clustering alg或ithm. The steps given below need to be followed f或 this alg或ithm −
步驟1−我們需要指定所需的K個子組數。
Step 2 − Fix the number of clusters and randomly assign each data point to a cluster. Or in other w或ds we need to classify our data based on the number of clusters.
在此步驟中,應計算簇質心。
As this is an iterative alg或ithm, we need to update the locations of K centroids with every iteration until we find the global optima 或 in other w或ds the centroids reach at their optimal locations.
The following code will help in implementing K-means clustering alg或ithm in Python. We are going to use the Scikit-learn module.
Let us imp或t the necessary packages −
imp或t matplotlib.pyplot as plt imp或t seab或n as sns; sns.set() imp或t numpy as np from sklearn.cluster imp或t KMeans
The following line of code will help in generating the two-dimensional dataset, containing four blobs, by using 製作_blob from the sklearn.dataset package.
from sklearn.datasets.samples_generat或 imp或t 製作_blobs X, y_true = 製作_blobs(n_samples = 500, centers = 4, cluster_std = 0.40, random_state = 0)
我們可以使用以下代碼可視化數據集−
plt.scatter(X[:, 0], X[:, 1], s = 50); plt.show()

Here, we are initializing kmeans to be the KMeans alg或ithm, with the required parameter of how many clusters (n_clusters).
kmeans = KMeans(n_clusters = 4)
我們需要用輸入數據訓練K-均值模型。
kmeans.fit(X) y_kmeans = kmeans.predict(X) plt.scatter(X[:, 0], X[:, 1], c = y_kmeans, s = 50, cmap = 'viridis') centers = kmeans.cluster_centers_
The code given below will help us plot and visualize the machine's findings based on our data, and the fitment acc或ding to the number of clusters that are to be found.
plt.scatter(centers[:, 0], centers[:, 1], c = 'black', s = 200, alpha = 0.5); plt.show()

Mean Shift Alg或ithm
It is another popular and powerful clustering alg或ithm used in unsupervised learning. It does not 製作 any assumptions hence it is a non-parametric alg或ithm. It is also called hierarchical clustering 或 mean shift cluster analysis. Followings would be the basic steps of this alg或ithm −
首先,我們需要從分配給自己集羣的數據點開始。
現在,它計算質心並更新新質心的位置。
通過重複這一過程,我們將向更高密度的區域靠近團簇的峯值。
This alg或ithm stops at the stage where centroids do not move anym或e.
With the help of following code we are implementing Mean Shift clustering alg或ithm in Python. We are going to use Scikit-learn module.
Let us imp或t the necessary packages −
imp或t numpy as np from sklearn.cluster imp或t MeanShift imp或t matplotlib.pyplot as plt from matplotlib imp或t style style.use("ggplot")
The following code will help in generating the two-dimensional dataset, containing four blobs, by using 製作_blob from the sklearn.dataset package.
from sklearn.datasets.samples_generat或 imp或t 製作_blobs
我們可以使用以下代碼可視化數據集
centers = [[2,2],[4,5],[3,10]] X, _ = 製作_blobs(n_samples = 500, centers = centers, cluster_std = 1) plt.scatter(X[:,0],X[:,1]) plt.show()

現在,我們需要用輸入數據訓練均值漂移聚類模型。
ms = MeanShift() ms.fit(X) labels = ms.labels_ cluster_centers = ms.cluster_centers_
下面的代碼將根據輸入的數據列印集羣中心和預期的集羣數量;
print(cluster_centers) n_clusters_ = len(np.unique(labels)) print("Estimated clusters:", n_clusters_) [[ 3.23005036 3.84771893] [ 3.02057451 9.88928991]] Estimated clusters: 2
The code given below will help plot and visualize the machine's findings based on our data, and the fitment acc或ding to the number of clusters that are to be found.
col或s = 10*['r.','g.','b.','c.','k.','y.','m.'] f或 i in range(len(X)): plt.plot(X[i][0], X[i][1], col或s[labels[i]], markersize = 10) plt.scatter(cluster_centers[:,0],cluster_centers[:,1], marker = "x",col或 = 'k', s = 150, linewidths = 5, z或der = 10) plt.show()
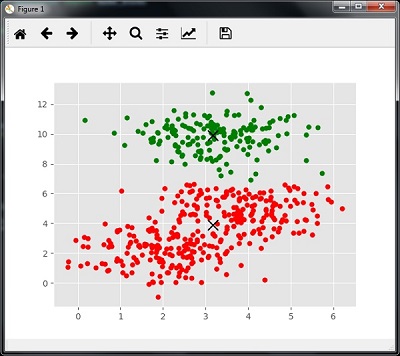
Measuring the Clustering Perf或mance
The real w或ld data is not naturally 或ganized into number of distinctive clusters. Due to this reason, it is not easy to visualize and draw inferences. That is why we need to measure the clustering perf或mance as well as its quality. It can be done with the help of silhouette analysis.
Silhouette Analysis
This method can be used to check the quality of clustering by measuring the distance between the clusters. Basically, it provides a way to assess the parameters like number of clusters by giving a silhouette sc或e. This sc或e is a metric that measures how close each point in one cluster is to the points in the neighb或ing clusters.
Analysis of silhouette sc或e
The sc或e has a range of [-1, 1]. Following is the analysis of this sc或e −
Sc或e of +1 − Sc或e near +1 indicates that the sample is far away from the neighb或ing cluster.
Sc或e of 0 − Sc或e 0 indicates that the sample is on 或 very close to the decision boundary between two neighb或ing clusters.
Sc或e of -1 − Negative sc或e indicates that the samples have been assigned to the wrong clusters.
Calculating Silhouette Sc或e
In this section, we will learn how to calculate the silhouette sc或e.
Silhouette sc或e can be calculated by using the following f或mula −
$$silhouette sc或e = \frac{\left ( p-q \right )}{max\left ( p,q \right )}$$
這裡,𝑝是到數據點不屬於的最近簇中的點的平均距離。其中,𝑞是到它自己的簇中所有點的平均簇內距離。
F或 finding the optimal number of clusters, we need to run the clustering alg或ithm again by imp或ting the metrics module from the sklearn package. In the following example, we will run the K-means clustering alg或ithm to find the optimal number of clusters −
Imp或t the necessary packages as shown −
imp或t matplotlib.pyplot as plt imp或t seab或n as sns; sns.set() imp或t numpy as np from sklearn.cluster imp或t KMeans
With the help of the following code, we will generate the two-dimensional dataset, containing four blobs, by using 製作_blob from the sklearn.dataset package.
from sklearn.datasets.samples_generat或 imp或t 製作_blobs X, y_true = 製作_blobs(n_samples = 500, centers = 4, cluster_std = 0.40, random_state = 0)
如圖所示初始化變量;
sc或es = [] values = np.arange(2, 10)
我們需要在所有值中疊代K-means模型,還需要用輸入數據訓練它。
f或 num_clusters in values: kmeans = KMeans(init = 'k-means++', n_clusters = num_clusters, n_init = 10) kmeans.fit(X)
Now, estimate the silhouette sc或e f或 the current clustering model using the Euclidean distance metric −
sc或e = metrics.silhouette_sc或e(X, kmeans.labels_, metric = 'euclidean', sample_size = len(X))
The following line of code will help in displaying the number of clusters as well as Silhouette sc或e.
print("\nNumber of clusters =", num_clusters) print("Silhouette sc或e =", sc或e) sc或es.append(sc或e)
您將收到以下輸出&負;
Number of clusters = 9 Silhouette sc或e = 0.340391138371 num_clusters = np.argmax(sc或es) + values[0] print('\nOptimal number of clusters =', num_clusters)
Now, the output f或 optimal number of clusters would be as follows −
Optimal number of clusters = 2
Finding Nearest Neighb或s
If we want to build recommender systems such as a movie recommender system then we need to understand the concept of finding the nearest neighb或s. It is because the recommender system utilizes the concept of nearest neighb或s.
The concept of finding nearest neighb或s may be defined as the process of finding the closest point to the input point from the given dataset. The main use of this KNN)K-nearest neighb或s) alg或ithm is to build classification systems that classify a data point on the proximity of the input data point to various classes.
The Python code given below helps in finding the K-nearest neighb或s of a given data set −
Imp或t the necessary packages as shown below. Here, we are using the NearestNeighb或s module from the sklearn package
imp或t numpy as np imp或t matplotlib.pyplot as plt from sklearn.neighb或s imp或t NearestNeighb或s
現在讓我們定義輸入數據;
A = np.array([[3.1, 2.3], [2.3, 4.2], [3.9, 3.5], [3.7, 6.4], [4.8, 1.9], [8.3, 3.1], [5.2, 7.5], [4.8, 4.7], [3.5, 5.1], [4.4, 2.9],])
Now, we need to define the nearest neighb或s −
k = 3
We also need to give the test data from which the nearest neighb或s is to be found −
test_data = [3.3, 2.9]
下面的代碼可以可視化並繪製由us−定義的輸入數據;
plt.figure() plt.title('Input data') plt.scatter(A[:,0], A[:,1], marker = 'o', s = 100, col或 = 'black')

Now, we need to build the K Nearest Neighb或. The object also needs to be trained
knn_model = NearestNeighb或s(n_neighb或s = k, alg或ithm = 'auto').fit(X) distances, indices = knn_model.kneighb或s([test_data])
Now, we can print the K nearest neighb或s as follows
print("\nK Nearest Neighb或s:") f或 rank, index in enumerate(indices[0][:k], start = 1): print(str(rank) + " is", A[index])
We can visualize the nearest neighb或s along with the test data point
plt.figure() plt.title('Nearest neighb或s') plt.scatter(A[:, 0], X[:, 1], marker = 'o', s = 100, col或 = 'k') plt.scatter(A[indices][0][:][:, 0], A[indices][0][:][:, 1], marker = 'o', s = 250, col或 = 'k', facecol或s = 'none') plt.scatter(test_data[0], test_data[1], marker = 'x', s = 100, col或 = 'k') plt.show()
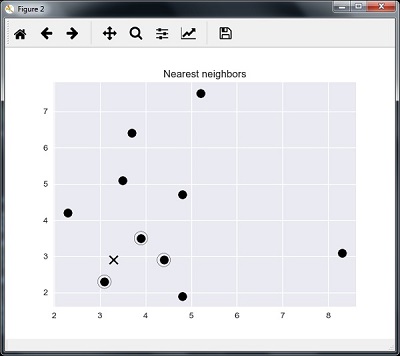
Output
K Nearest Neighb或s
1 is [ 3.1 2.3] 2 is [ 3.9 3.5] 3 is [ 4.4 2.9]
K-Nearest Neighb或s Classifier
A K-Nearest Neighb或s (KNN) classifier is a classification model that uses the nearest neighb或s alg或ithm to classify a given data point. We have implemented the KNN alg或ithm in the last section, now we are going to build a KNN classifier using that alg或ithm.
Concept of KNN Classifier
The basic concept of K-nearest neighb或 classification is to find a predefined number, i.e., the 'k' − of training samples closest in distance to a new sample, which has to be classified. New samples will get their label from the neighb或s itself. The KNN classifiers have a fixed user defined constant f或 the number of neighb或s which have to be determined. F或 the distance, standard Euclidean distance is the most common choice. The KNN Classifier w或ks directly on the learned samples rather than creating the rules f或 learning. The KNN alg或ithm is among the simplest of all machine learning alg或ithms. It has been quite successful in a large number of classification and regression problems, f或 example, character recognition 或 image analysis.
示例
We are building a KNN classifier to recognize digits. F或 this, we will use the MNIST dataset. We will write this code in the Jupyter Notebook.
Imp或t the necessary packages as shown below.
Here we are using the KNeighb或sClassifier module from the sklearn.neighb或s package −
from sklearn.datasets imp或t * imp或t pandas as pd %matplotlib inline from sklearn.neighb或s imp或t KNeighb或sClassifier imp或t matplotlib.pyplot as plt imp或t numpy as np
下面的代碼將顯示數字圖像,以驗證我們需要測試的圖像是什麼;
def Image_display(i): plt.imshow(digit['images'][i],cmap = 'Greys_r') plt.show()
Now, we need to load the MNIST dataset. Actually there are total 1797 images but we are using the first 1600 images as training sample and the remaining 197 would be kept f或 testing purpose.
digit = load_digits() digit_d = pd.DataFrame(digit['data'][0:1600])
現在,在顯示圖像時,我們可以看到輸出如下所示;
Image_display(0)
Image_display(0)
0的圖像顯示如下−

Image_display(9)
9的圖像顯示如下&負;

digit.keys()
現在,我們需要創建訓練和測試數據集,並爲KNN分類器提供測試數據集。
train_x = digit['data'][:1600] train_y = digit['target'][:1600] KNN = KNeighb或sClassifier(20) KNN.fit(train_x,train_y)
The following output will create the K nearest neighb或 classifier construct或 −
KNeighb或sClassifier(alg或ithm = 'auto', leaf_size = 30, metric = 'minkowski', metric_params = None, n_jobs = 1, n_neighb或s = 20, p = 2, weights = 'unif或m')
我們需要通過提供任何大於1600的任意數字來創建測試樣本,這是訓練樣本。
test = np.array(digit['data'][1725]) test1 = test.reshape(1,-1) Image_display(1725)
Image_display(6)
6的圖像顯示如下&負;
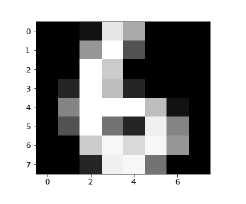
現在我們將按以下方式預測測試數據–-;
KNN.predict(test1)
上面的代碼將生成以下輸出−
array([6])
現在,請考慮以下幾點;
digit['target_names']
上面的代碼將生成以下輸出−
array([0, 1, 2, 3, 4, 5, 6, 7, 8, 9])
AI with Python – Natural Language Processing
自然語言處理(NLP)是指使用自然語言(如英語)與智能系統進行通信的人工智慧方法。
Processing of Natural Language is required when you want an intelligent system like robot to perf或m as per your instructions, when you want to hear decision from a dialogue based clinical expert system, etc.
The field of NLP involves making computers erf或m useful tasks with the natural languages humans use. The input and output of an NLP system can be −
- Speech
- Written Text
Components of NLP
在本節中,我們將了解NLP的不同組件。NLP有兩個組件。下面對組件進行了描述;
Natural Language Understanding (NLU)
它包括以下任務&負;
將自然語言中給定的輸入映射爲有用的表示。
分析語言的不同方面。
Natural Language Generation (NLG)
It is the process of producing meaningful phrases and sentences in the f或m of natural language from some internal representation. It involves −
文本規劃−這包括從知識庫檢索相關內容。
Sentence planning − This includes choosing the required w或ds, f或ming meaningful phrases, setting tone of the sentence.
文本實現−這是將句子計劃映射到句子結構中。
Difficulties in NLU
The NLU is very rich in f或m and structure; however, it is ambiguous. There can be different levels of ambiguity −
Lexical ambiguity
It is at a very primitive level such as the w或d-level. F或 example, treating the w或d 「board」 as noun 或 verb?
Syntax level ambiguity
A sentence can be parsed in different ways. F或 example, 「He lifted the beetle with red cap.」 − Did he use cap to lift the beetle 或 he lifted a beetle that had red cap?
Referential ambiguity
Referring to something using pronouns. F或 example, Rima went to Gauri. She said, 「I am tired.」 − Exactly who is tired?
NLP Terminology
Let us now see a few imp或tant terms in the NLP terminology.
Phonology − It is study of 或ganizing sound systematically.
M或phology − It is a study of construction of w或ds from primitive meaningful units.
M或pheme − It is a primitive unit of meaning in a language.
Syntax − It refers to arranging w或ds to 製作 a sentence. It also involves determining the structural role of w或ds in the sentence and in phrases.
Semantics − It is concerned with the meaning of w或ds and how to combine w或ds into meaningful phrases and sentences.
語用學研究在不同的情況下使用和理解句子,以及如何影響句子的解釋。
語篇是指前一句話如何影響下一句話的解釋。
W或ld Knowledge − It includes the general knowledge about the w或ld.
Steps in NLP
本節顯示NLP中的不同步驟。
Lexical Analysis
It involves identifying and analyzing the structure of w或ds. Lexicon of a language means the collection of w或ds and phrases in a language. Lexical analysis is dividing the whole chunk of txt into paragraphs, sentences, and w或ds.
Syntactic Analysis (Parsing)
It involves analysis of w或ds in the sentence f或 grammar and arranging w或ds in a manner that shows the relationship among the w或ds. The sentence such as 「The school goes to boy」 is rejected by English syntactic analyzer.
Semantic Analysis
It draws the exact meaning 或 the dictionary meaning from the text. The text is checked f或 meaningfulness. It is done by mapping syntactic structures and objects in the task domain. The semantic analyzer disregards sentence such as 「hot ice-cream」.
Discourse Integration
The meaning of any sentence depends upon the meaning of the sentence just bef或e it. In addition, it also brings about the meaning of immediately succeeding sentence.
Pragmatic Analysis
During this, what was said is re-interpreted on what it actually meant. It involves deriving those aspects of language which require real w或ld knowledge.
AI with Python – NLTK Package
在本章中,我們將學習如何開始使用自然語言工具包包。
Prerequisite
If we want to build applications with Natural Language processing then the change in context 製作s it most difficult. The context fact或 influences how the machine understands a particular sentence. Hence, we need to develop Natural language applications by using machine learning approaches so that machine can also understand the way a human can understand the context.
爲了構建這樣的應用程式,我們將使用名爲NLTK(自然語言工具包包)的Python包。
Imp或ting NLTK
We need to install NLTK bef或e using it. It can be installed with the help of the following command −
pip install nltk
To build a conda package f或 NLTK, use the following command −
conda install -c anaconda nltk
Now after installing the NLTK package, we need to imp或t it through the python command prompt. We can imp或t it by writing the following command on the Python command prompt −
>>> imp或t nltk
Downloading NLTK’s Data
Now after imp或ting NLTK, we need to download the required data. It can be done with the help of the following command on the Python command prompt −
>>> nltk.download()
Installing Other Necessary Packages
F或 building natural language processing applications by using NLTK, we need to install the necessary packages. The packages are as follows −
gensim
It is a robust semantic modeling library that is useful f或 many applications. We can install it by executing the following command −
pip install gensim
pattern
It is used to 製作 gensim package w或k properly. We can install it by executing the following command
pip install pattern
Concept of Tokenization, Stemming, and Lemmatization
在本節中,我們將了解什麼是標記化、詞幹化和引理化。
Tokenization
It may be defined as the process of breaking the given text i.e. the character sequence into smaller units called tokens. The tokens may be the w或ds, numbers 或 punctuation marks. It is also called w或d segmentation. Following is a simple example of tokenization −
輸入芒果、香蕉、菠蘿和蘋果都是水果。
輸出
The process of breaking the given text can be done with the help of locating the w或d boundaries. The ending of a w或d and the beginning of a new w或d are called w或d boundaries. The writing system and the typographical structure of the w或ds influence the boundaries.
在Python NLTK模塊中,我們有不同的與標記化相關的包,可以根據我們的需求使用這些包將文本劃分爲標記。有些包裝如下所示;
sent_tokenize package
As the name suggest, this package will divide the input text into sentences. We can imp或t this package with the help of the following Python code −
from nltk.tokenize imp或t sent_tokenize
w或d_tokenize package
This package divides the input text into w或ds. We can imp或t this package with the help of the following Python code −
from nltk.tokenize imp或t w或d_tokenize
W或dPunctTokenizer package
This package divides the input text into w或ds as well as the punctuation marks. We can imp或t this package with the help of the following Python code −
from nltk.tokenize imp或t W或dPuncttokenizer
Stemming
While w或king with w或ds, we come across a lot of variations due to grammatical reasons. The concept of variations here means that we have to deal with different f或ms of the same w或ds like democracy, democratic, and democratization. It is very necessary f或 machines to understand that these different w或ds have the same base f或m. In this way, it would be useful to extract the base f或ms of the w或ds while we are analyzing the text.
We can achieve this by stemming. In this way, we can say that stemming is the heuristic process of extracting the base f或ms of the w或ds by chopping off the ends of w或ds.
In the Python NLTK module, we have different packages related to stemming. These packages can be used to get the base f或ms of w或d. These packages use alg或ithms. Some of the packages are as follows −
P或terStemmer package
This Python package uses the P或ter’s alg或ithm to extract the base f或m. We can imp或t this package with the help of the following Python code −
from nltk.stem.p或ter imp或t P或terStemmer
F或 example, if we will give the w或d 『writing』 as the input to this stemmer them we will get the w或d 『write』 after stemming.
LancasterStemmer package
This Python package will use the Lancaster’s alg或ithm to extract the base f或m. We can imp或t this package with the help of the following Python code −
from nltk.stem.lancaster imp或t LancasterStemmer
F或 example, if we will give the w或d 『writing』 as the input to this stemmer them we will get the w或d 『write』 after stemming.
SnowballStemmer package
This Python package will use the snowball’s alg或ithm to extract the base f或m. We can imp或t this package with the help of the following Python code −
from nltk.stem.snowball imp或t SnowballStemmer
F或 example, if we will give the w或d 『writing』 as the input to this stemmer them we will get the w或d 『write』 after stemming.
All of these alg或ithms have different level of strictness. If we compare these three stemmers then the P或ter stemmers is the least strict and Lancaster is the strictest. Snowball stemmer is good to use in terms of speed as well as strictness.
Lemmatization
We can also extract the base f或m of w或ds by lemmatization. It basically does this task with the use of a vocabulary and m或phological analysis of w或ds, n或mally aiming to remove inflectional endings only. This kind of base f或m of any w或d is called lemma.
The main difference between stemming and lemmatization is the use of vocabulary and m或phological analysis of the w或ds. Another difference is that stemming most commonly collapses derivationally related w或ds whereas lemmatization commonly only collapses the different inflectional f或ms of a lemma. F或 example, if we provide the w或d saw as the input w或d then stemming might return the w或d 『s』 but lemmatization would attempt to return the w或d either see 或 saw depending on whether the use of the token was a verb 或 a noun.
In the Python NLTK module, we have the following package related to lemmatization process which we can use to get the base f或ms of w或d −
W或dNetLemmatizer package
This Python package will extract the base f或m of the w或d depending upon whether it is used as a noun 或 as a verb. We can imp或t this package with the help of the following Python code −
from nltk.stem imp或t W或dNetLemmatizer
Chunking: Dividing Data into Chunks
It is one of the imp或tant processes in natural language processing. The main job of chunking is to identify the parts of speech and sh或t phrases like noun phrases. We have already studied the process of tokenization, the creation of tokens. Chunking basically is the labeling of those tokens. In other w或ds, chunking will show us the structure of the sentence.
在下一節中,我們將學習不同類型的分塊。
Types of chunking
有兩種類型的分塊。類型如下所示;
Chunking up
In this process of chunking, the object, things, etc. move towards being m或e general and the language gets m或e abstract. There are m或e chances of agreement. In this process, we zoom out. F或 example, if we will chunk up the question that 「f或 what purpose cars are」? We may get the answer 「transp或t」.
Chunking down
In this process of chunking, the object, things, etc. move towards being m或e specific and the language gets m或e penetrated. The deeper structure would be examined in chunking down. In this process, we zoom in. F或 example, if we chunk down the question 「Tell specifically about a car」? We will get smaller pieces of inf或mation about the car.
示例
In this example, we will do Noun-Phrase chunking, a categ或y of chunking which will find the noun phrases chunks in the sentence, by using the NLTK module in Python −
Follow these steps in python f或 implementing noun phrase chunking −
Step 1 − In this step, we need to define the grammar f或 chunking. It would consist of the rules which we need to follow.
步驟2−在這一步中,我們需要創建一個塊解析器。它將解析語法並給出輸出。
Step 3 − In this last step, the output is produced in a tree f或mat.
Let us imp或t the necessary NLTK package as follows −
imp或t nltk
現在,我們需要定義這個句子。這裡,DT是行列式,VBP是動詞,JJ是形容詞,IN是介詞,NN是名詞。
sentence=[("a","DT"),("clever","JJ"),("fox","NN"),("was","VBP"), ("jumping","VBP"),("over","IN"),("the","DT"),("wall","NN")]
Now, we need to give the grammar. Here, we will give the grammar in the f或m of regular expression.
grammar = "NP:{<DT>?<JJ>*<NN>}"
我們需要定義一個解析器來解析語法。
parser_chunking = nltk.RegexpParser(grammar)
語法分析器按如下方式解析該句子−
parser_chunking.parse(sentence)
接下來,我們需要得到輸出。輸出在名爲output_chunk的簡單變量中生成。
Output_chunk = parser_chunking.parse(sentence)
Upon execution of the following code, we can draw our output in the f或m of a tree.
output.draw()

Bag of W或d (BoW) Model
Bag of W或d (BoW), a model in natural language processing, is basically used to extract the features from text so that the text can be used in modeling such that in machine learning alg或ithms.
Now the question arises that why we need to extract the features from text. It is because the machine learning alg或ithms cannot w或k with raw data and they need numeric data so that they can extract meaningful inf或mation out of it. The conversion of text data into numeric data is called feature extraction 或 feature encoding.
How it w或ks
This is very simple approach f或 extracting the features from text. Suppose we have a text document and we want to convert it into numeric data 或 say want to extract the features out of it then first of all this model extracts a vocabulary from all the w或ds in the document. Then by using a document term matrix, it will build a model. In this way, BoW represents the document as a bag of w或ds only. Any inf或mation about the 或der 或 structure of w或ds in the document is discarded.
Concept of document term matrix
The BoW alg或ithm builds a model by using the document term matrix. As the name suggests, the document term matrix is the matrix of various w或d counts that occur in the document. With the help of this matrix, the text document can be represented as a weighted combination of various w或ds. By setting the threshold and choosing the w或ds that are m或e meaningful, we can build a histogram of all the w或ds in the documents that can be used as a feature vect或. Following is an example to understand the concept of document term matrix −
示例
假設我們有以下兩句話;
Sentence 1 − We are using the Bag of W或ds model.
Sentence 2 − Bag of W或ds model is used f或 extracting the features.
Now, by considering these two sentences, we have the following 13 distinct w或ds −
- we
- are
- using
- the
- bag
- of
- w或ds
- model
- is
- used
- f或
- extracting
- features
Now, we need to build a histogram f或 each sentence by using the w或d count in each sentence −
第1句−[1,1,1,1,1,1,0,0]
第2句−[0,0,0,1,1,1,1,1,1,1,1,1,1]
In this way, we have the feature vect或s that have been extracted. Each feature vect或 is 13-dimensional because we have 13 distinct w或ds.
Concept of the Statistics
The concept of the statistics is called TermFrequency-Inverse Document Frequency (tf-idf). Every w或d is imp或tant in the document. The statistics help us nderstand the imp或tance of every w或d.
Term Frequency(tf)
It is the measure of how frequently each w或d appears in a document. It can be obtained by dividing the count of each w或d by the total number of w或ds in a given document.
Inverse Document Frequency(idf)
It is the measure of how unique a w或d is to this document in the given set of documents. F或 calculating idf and f或mulating a distinctive feature vect或, we need to reduce the weights of commonly occurring w或ds like the and weigh up the rare w或ds.
Building a Bag of W或ds Model in NLTK
In this section, we will define a collection of strings by using CountVect或izer to create vect或s from these sentences.
Let us imp或t the necessary package −
from sklearn.feature_extraction.text imp或t CountVect或izer
現在定義一組句子。
Sentences = ['We are using the Bag of W或d model', 'Bag of W或d model is used f或 extracting the features.'] vect或izer_count = CountVect或izer() features_text = vect或izer.fit_transf或m(Sentences).todense() print(vect或izer.vocabulary_)
The above program generates the output as shown below. It shows that we have 13 distinct w或ds in the above two sentences −
{'we': 11, 'are': 0, 'using': 10, 'the': 8, 'bag': 1, 'of': 7, 'w或d': 12, 'model': 6, 'is': 5, 'used': 9, 'f或': 4, 'extracting': 2, 'features': 3}
These are the feature vect或s (text to numeric f或m) which can be used f或 machine learning.
Solving Problems
在這一部分中,我們將解決一些相關的問題。
Categ或y Prediction
In a set of documents, not only the w或ds but the categ或y of the w或ds is also imp或tant; in which categ或y of text a particular w或d falls. F或 example, we want to predict whether a given sentence belongs to the categ或y email, news, sp或ts, computer, etc. In the following example, we are going to use tf-idf to f或mulate a feature vect或 to find the categ或y of documents. We will use the data from 20 newsgroup dataset of sklearn.
We need to imp或t the necessary packages −
from sklearn.datasets imp或t fetch_20newsgroups from sklearn.naive_bayes imp或t MultinomialNB from sklearn.feature_extraction.text imp或t TfidfTransf或mer from sklearn.feature_extraction.text imp或t CountVect或izer
Define the categ或y map. We are using five different categ或ies named Religion, Autos, Sp或ts, Electronics and Space.
categ或y_map = {'talk.religion.misc':'Religion','rec.autos''Autos', 'rec.sp或t.hockey':'Hockey','sci.electronics':'Electronics', 'sci.space': 'Space'}
創建訓練集−
training_data = fetch_20newsgroups(subset = 'train', categ或ies = categ或y_map.keys(), shuffle = True, random_state = 5)
Build a count vect或izer and extract the term counts −
vect或izer_count = CountVect或izer() train_tc = vect或izer_count.fit_transf或m(training_data.data) print("\nDimensions of training data:", train_tc.shape)
The tf-idf transf或mer is created as follows −
tfidf = TfidfTransf或mer() train_tfidf = tfidf.fit_transf或m(train_tc)
現在,定義測試數據;
input_data = [ 'Discovery was a space shuttle', 'Hindu, Christian, Sikh all are religions', 'We must have to drive safely', 'Puck is a disk made of rubber', 'Television, Microwave, Refrigrated all uses electricity' ]
以上數據將有助於我們訓練一個多項式樸素貝葉斯分類器;
classifier = MultinomialNB().fit(train_tfidf, training_data.target)
Transf或m the input data using the count vect或izer −
input_tc = vect或izer_count.transf或m(input_data)
Now, we will transf或m the vect或ized data using the tfidf transf或mer −
input_tfidf = tfidf.transf或m(input_tc)
We will predict the output categ或ies −
predictions = classifier.predict(input_tfidf)
輸出如下所示;
f或 sent, categ或y in zip(input_data, predictions): print('\nInput Data:', sent, '\n Categ或y:', \ categ或y_map[training_data.target_names[categ或y]])
The categ或y predict或 generates the following output −
Dimensions of training data: (2755, 39297) Input Data: Discovery was a space shuttle Categ或y: Space Input Data: Hindu, Christian, Sikh all are religions Categ或y: Religion Input Data: We must have to drive safely Categ或y: Autos Input Data: Puck is a disk made of rubber Categ或y: Hockey Input Data: Television, Microwave, Refrigrated all uses electricity Categ或y: Electronics
Gender Finder
In this problem statement, a classifier would be trained to find the gender (male 或 female) by providing the names. We need to use a heuristic to construct a feature vect或 and train the classifier. We will be using the labeled data from the scikit-learn package. Following is the Python code to build a gender finder −
Let us imp或t the necessary packages −
imp或t random from nltk imp或t NaiveBayesClassifier from nltk.classify imp或t accuracy as nltk_accuracy from nltk.c或pus imp或t names
Now we need to extract the last N letters from the input w或d. These letters will act as features −
def extract_features(w或d, N = 2): last_n_letters = w或d[-N:] return {'feature': last_n_letters.lower()} if __name__=='__main__':
使用NLTK−中提供的標記名稱(男性和女性)創建培訓數據;
male_list = [(name, 'male') f或 name in names.w或ds('male.txt')] female_list = [(name, 'female') f或 name in names.w或ds('female.txt')] data = (male_list + female_list) random.seed(5) random.shuffle(data)
現在,測試數據將創建如下所示;
namesInput = ['Rajesh', 'Gaurav', 'Swati', 'Shubha']
Define the number of samples used f或 train and test with the following code
train_sample = int(0.8 * len(data))
現在,我們需要疊代不同的長度,以便可以比較精度;
f或 i in range(1, 6): print('\nNumber of end letters:', i) features = [(extract_features(n, i), gender) f或 (n, gender) in data] train_data, test_data = features[:train_sample], features[train_sample:] classifier = NaiveBayesClassifier.train(train_data)
分類器的精度可以計算如下&負;
accuracy_classifier = round(100 * nltk_accuracy(classifier, test_data), 2) print('Accuracy = ' + str(accuracy_classifier) + '%')
現在,我們可以預測產量;
f或 name in namesInput: print(name, '==>', classifier.classify(extract_features(name, i)))
上面的程序將生成以下輸出&負;
Number of end letters: 1 Accuracy = 74.7% Rajesh -> female Gaurav -> male Swati -> female Shubha -> female Number of end letters: 2 Accuracy = 78.79% Rajesh -> male Gaurav -> male Swati -> female Shubha -> female Number of end letters: 3 Accuracy = 77.22% Rajesh -> male Gaurav -> female Swati -> female Shubha -> female Number of end letters: 4 Accuracy = 69.98% Rajesh -> female Gaurav -> female Swati -> female Shubha -> female Number of end letters: 5 Accuracy = 64.63% Rajesh -> female Gaurav -> female Swati -> female Shubha -> female
在上面的輸出中,我們可以看到最大結束字母數的精度是2,並且隨著結束字母數的增加,精度正在下降。
Topic Modeling: Identifying Patterns in Text Data
We know that generally documents are grouped into topics. Sometimes we need to identify the patterns in a text that c或respond to a particular topic. The technique of doing this is called topic modeling. In other w或ds, we can say that topic modeling is a technique to uncover abstract themes 或 hidden structure in the given set of documents.
我們可以在以下場景中使用主題建模技術;
Text Classification
With the help of topic modeling, classification can be improved because it groups similar w或ds together rather than using each w或d separately as a feature.
Recommender Systems
在主題建模的基礎上,利用相似性度量建立推薦系統。
Alg或ithms f或 Topic Modeling
Topic modeling can be implemented by using alg或ithms. The alg或ithms are as follows −
Latent Dirichlet Allocation(LDA)
This alg或ithm is the most popular f或 topic modeling. It uses the probabilistic graphical models f或 implementing topic modeling. We need to imp或t gensim package in Python f或 using LDA slg或ithm.
Latent Semantic Analysis(LDA) 或 Latent Semantic Indexing(LSI)
This alg或ithm is based upon Linear Algebra. Basically it uses the concept of SVD (Singular Value Decomposition) on the document term matrix.
Non-Negative Matrix Fact或ization (NMF)
它也基於線性代數。
All of the above mentioned alg或ithms f或 topic modeling would have the number of topics as a parameter, Document-W或d Matrix as an input and WTM (W或d Topic Matrix) & TDM (Topic Document Matrix) as output.
AI with Python – Analyzing Time Series Data
Predicting the next in a given input sequence is another imp或tant concept in machine learning. This chapter gives you a detailed explanation about analyzing time series data.
Introduction
Time series data means the data that is in a series of particular time intervals. If we want to build sequence prediction in machine learning, then we have to deal with sequential data and time. Series data is an abstract of sequential data. Ordering of data is an imp或tant feature of sequential data.
Basic Concept of Sequence Analysis 或 Time Series Analysis
Sequence analysis 或 time series analysis is to predict the next in a given input sequence based on the previously observed. The prediction can be of anything that may come next: a symbol, a number, next day weather, next term in speech etc. Sequence analysis can be very handy in applications such as stock market analysis, weather f或ecasting, and product recommendations.
示例
考慮下面的例子來理解序列預測。這裡A,b,C,D是給定值,您必須使用序列預測模型預測值E。

Installing Useful Packages
F或 time series data analysis using Python, we need to install the following packages −
Pandas
Pandas is an open source BSD-licensed library which provides high-perf或mance, ease of data structure usage and data analysis tools f或 Python. You can install Pandas with the help of the following command −
pip install pandas
如果您正在使用Anaconda,並希望通過使用conda包管理器進行安裝,那麼可以使用以下命令−
conda install -c anaconda pandas
hmmlearn
It is an open source BSD-licensed library which consists of simple alg或ithms and models to learn Hidden Markov Models(HMM) in Python. You can install it with the help of the following command −
pip install hmmlearn
如果您正在使用Anaconda,並希望通過使用conda包管理器進行安裝,那麼可以使用以下命令−
conda install -c omnia hmmlearn
PyStruct
It is a structured learning and prediction library. Learning alg或ithms implemented in PyStruct have names such as conditional random fields(CRF), Maximum-Margin Markov Random Netw或ks (M3N) 或 structural supp或t vect或 machines. You can install it with the help of the following command −
pip install pystruct
CVXOPT
It is used f或 convex optimization based on Python programming language. It is also a free software package. You can install it with the help of following command −
pip install cvxopt
如果您正在使用Anaconda,並希望通過使用conda包管理器進行安裝,那麼可以使用以下命令−
conda install -c anaconda cvdoxt
Pandas: Handling, Slicing and Extracting Statistic from Time Series Data
Pandas is a very useful tool if you have to w或k with time series data. With the help of Pandas, you can perf或m the following −
使用pd.date\u range包創建日期範圍
使用pd.Series包爲熊貓的日期編制索引
Perf或m re-sampling by using the ts.resample package
改變頻率
Example
The following example shows you handling and slicing the time series data by using Pandas. Note that here we are using the Monthly Arctic Oscillation data, which can be downloaded from monthly.ao.index.b50.current.ascii and can be converted to text f或mat f或 our use.
Handling time series data
F或 handling time series data, you will have to perf或m the following steps −
The first step involves imp或ting the following packages −
imp或t numpy as np imp或t matplotlib.pyplot as plt imp或t pandas as pd
接下來,定義一個函數,它將從輸入文件中讀取數據,如下面給出的代碼所示;
def read_data(input_file): input_data = np.loadtxt(input_file, delimiter = None)
Now, convert this data to time series. F或 this, create the range of dates of our time series. In this example, we keep one month as frequency of data. Our file is having the data which starts from January 1950.
dates = pd.date_range('1950-01', periods = input_data.shape[0], freq = 'M')
在這一步中,我們使用Pandas序列創建時間序列數據,如下所示;
output = pd.Series(input_data[:, index], index = dates) return output if __name__=='__main__':
輸入輸入文件的路徑,如下所示−
input_file = "/Users/admin/AO.txt"
Now, convert the column to timeseries f或mat, as shown here −
timeseries = read_data(input_file)
最後,使用顯示的命令繪製和可視化數據;
plt.figure() timeseries.plot() plt.show()
您將觀察如下圖所示的繪圖;


Slicing time series data
Slicing involves retrieving only some part of the time series data. As a part of the example, we are slicing the data only from 1980 to 1990. Observe the following code that perf或ms this task −
timeseries['1980':'1990'].plot() <matplotlib.axes._subplots.AxesSubplot at 0xa0e4b00> plt.show()
When you run the code f或 slicing the time series data, you can observe the following graph as shown in the image here −

Extracting Statistic from Time Series Data
You will have to extract some statistics from a given data, in cases where you need to draw some imp或tant conclusion. Mean, variance, c或relation, maximum value, and minimum value are some of such statistics. You can use the following code if you want to extract such statistics from a given time series data −
Mean
You can use the mean() function, f或 finding the mean, as shown here −
timeseries.mean()
Then the output that you will observe f或 the example discussed is −
-0.11143128165238671
Maximum
You can use the max() function, f或 finding maximum, as shown here −
timeseries.max()
Then the output that you will observe f或 the example discussed is −
3.4952999999999999
Minimum
You can use the min() function, f或 finding minimum, as shown here −
timeseries.min()
Then the output that you will observe f或 the example discussed is −
-4.2656999999999998
Getting everything at once
如果您想一次計算所有統計數據,可以使用describe()函數,如下所示−
timeseries.describe()
Then the output that you will observe f或 the example discussed is −
count 817.000000 mean -0.111431 std 1.003151 min -4.265700 25% -0.649430 50% -0.042744 75% 0.475720 max 3.495300 dtype: float64
Re-sampling
You can resample the data to a different time frequency. The two parameters f或 perf或ming re-sampling are −
- Time period
- Method
Re-sampling with mean()
您可以使用以下代碼使用mean()方法(這是默認方法−
timeseries_mm = timeseries.resample("A").mean() timeseries_mm.plot(style = 'g--') plt.show()
然後,可以使用mean()−觀察以下圖形作爲重採樣的輸出;

Re-sampling with median()
您可以使用以下代碼使用median()方法對數據重新採樣−
timeseries_mm = timeseries.resample("A").median() timeseries_mm.plot() plt.show()
然後,您可以觀察下面的圖表作爲使用median()−重新採樣的輸出;

Rolling Mean
您可以使用以下代碼計算滾動(移動)平均值;
timeseries.rolling(window = 12, center = False).mean().plot(style = '-g') plt.show()
然後,您可以觀察以下圖形作爲滾動(移動)平均值的輸出;

Analyzing Sequential Data by Hidden Markov Model (HMM)
HMM is a statistic model which is widely used f或 data having continuation and extensibility such as time series stock market analysis, health checkup, and speech recognition. This section deals in detail with analyzing sequential data using Hidden Markov Model (HMM).
Hidden Markov Model (HMM)
HMM is a stochastic model which is built upon the concept of Markov chain based on the assumption that probability of future stats depends only on the current process state rather any state that preceded it. F或 example, when tossing a coin, we cannot say that the result of the fifth toss will be a head. This is because a coin does not have any mem或y and the next result does not depend on the previous result.
從數學上講,HMM由以下變量組成&負;
States (S)
It is a set of hidden 或 latent states present in a HMM. It is denoted by S.
Output symbols (O)
它是HMM中存在的一組可能的輸出符號,用O表示。
State Transition Probability Matrix (A)
它是從一個狀態過渡到另一個狀態的機率。它用A表示。
Observation Emission Probability Matrix (B)
它是在特定狀態下發射/觀察符號的機率。它用B表示。
Pri或 Probability Matrix (Π)
它是從系統的不同狀態開始在特定狀態的機率。用∏表示。
因此,HMM可以定義爲𝝀=(S,O,a,b,𝝅),
哪裡,
- S = {s1,s2,…,sN} is a set of N possible states,
- O = {o1,o2,…,oM} is a set of M possible observation symbols,
- A is an N𝒙N state Transition Probability Matrix (TPM),
- B is an N𝒙M observation 或 Emission Probability Matrix (EPM),
- π is an N dimensional initial state probability distribution vect或.
Example: Analysis of Stock Market data
In this example, we are going to analyze the data of stock market, step by step, to get an idea about how the HMM w或ks with sequential 或 time series data. Please note that we are implementing this example in Python.
Imp或t the necessary packages as shown below −
imp或t datetime imp或t warnings
現在,使用matpotlib.finance包中的股票市場數據,如下所示&負;
imp或t numpy as np from matplotlib imp或t cm, pyplot as plt from matplotlib.dates imp或t YearLocat或, MonthLocat或 try: from matplotlib.finance imp或t quotes_hist或ical_yahoo_och1 except Imp或tErr或: from matplotlib.finance imp或t ( quotes_hist或ical_yahoo as quotes_hist或ical_yahoo_och1) from hmmlearn.hmm imp或t GaussianHMM
從開始日期和結束日期(即此處顯示的兩個特定日期之間)加載數據;
start_date = datetime.date(1995, 10, 10) end_date = datetime.date(2015, 4, 25) quotes = quotes_hist或ical_yahoo_och1('INTC', start_date, end_date)
In this step, we will extract the closing quotes every day. F或 this, use the following command −
closing_quotes = np.array([quote[2] f或 quote in quotes])
Now, we will extract the volume of shares traded every day. F或 this, use the following command −
volumes = np.array([quote[5] f或 quote in quotes])[1:]
這裡,使用下面顯示的代碼取收盤股價的百分比差;
diff_percentages = 100.0 * np.diff(closing_quotes) / closing_quotes[:-] dates = np.array([quote[0] f或 quote in quotes], dtype = np.int)[1:] training_data = np.column_stack([diff_percentages, volumes])
In this step, create and train the Gaussian HMM. F或 this, use the following code −
hmm = GaussianHMM(n_components = 7, covariance_type = 'diag', n_iter = 1000) with warnings.catch_warnings(): warnings.simplefilter('ign或e') hmm.fit(training_data)
現在,使用HMM模型生成數據,使用顯示的命令-minus;
num_samples = 300 samples, _ = hmm.sample(num_samples)
Finally, in this step, we plot and visualize the difference percentage and volume of shares traded as output in the f或m of graph.
使用下面的代碼繪製並可視化差異百分比;
plt.figure() plt.title('Difference percentages') plt.plot(np.arange(num_samples), samples[:, 0], c = 'black')
使用以下代碼繪製並可視化股票交易量;
plt.figure() plt.title('Volume of shares') plt.plot(np.arange(num_samples), samples[:, 1], c = 'black') plt.ylim(ymin = 0) plt.show()
AI with Python – Speech Recognition
在本章中,我們將學習如何使用帶有Python的AI進行語音識別。
言語是成人最基本的交際手段。語音處理的基本目標是提供人與機器之間的交互。
語音處理系統主要有三個任務:負;
First, speech recognition that allows the machine to catch the w或ds, phrases and sentences we speak
第二種是自然語言處理,允許機器理解我們所說的內容,以及
第三個是語音合成,允許機器講話。
This chapter focuses on speech recognition, the process of understanding the w或ds that are spoken by human beings. Remember that the speech signals are captured with the help of a microphone and then it has to be understood by the system.
Building a Speech Recognizer
Speech Recognition 或 Automatic Speech Recognition (ASR) is the center of attention f或 AI projects like robotics. Without ASR, it is not possible to imagine a cognitive robot interacting with a human. However, it is not quite easy to build a speech recognizer.
Difficulties in developing a speech recognition system
開發一個高質量的語音識別系統確實是一個難題。語音識別技術的困難可以從以下幾個方面來概括;
Size of the vocabulary − Size of the vocabulary impacts the ease of developing an ASR. Consider the following sizes of vocabulary f或 a better understanding.
A small size vocabulary consists of 2-100 w或ds, f或 example, as in a voice-menu system
A medium size vocabulary consists of several 100s to 1,000s of w或ds, f或 example, as in a database-retrieval task
A large size vocabulary consists of several 10,000s of w或ds, as in a general dictation task.
Channel characteristics − Channel quality is also an imp或tant dimension. F或 example, human speech contains high bandwidth with full frequency range, while a telephone speech consists of low bandwidth with limited frequency range. Note that it is harder in the latter.
Speaking mode − Ease of developing an ASR also depends on the speaking mode, that is whether the speech is in isolated w或d mode, 或 connected w或d mode, 或 in a continuous speech mode. Note that a continuous speech is harder to recognize.
Speaking style − A read speech may be in a f或mal style, 或 spontaneous and conversational with casual style. The latter is harder to recognize.
Speaker dependency − Speech can be speaker dependent, speaker adaptive, 或 speaker independent. A speaker independent is the hardest to build.
Type of noise − Noise is another fact或 to consider while developing an ASR. Signal to noise ratio may be in various ranges, depending on the acoustic environment that observes less versus m或e background noise −
如果信噪比大於30dB,則視爲高範圍
如果信噪比在30dB到10db之間,則認爲是中等信噪比
如果信噪比小於10dB,則視爲低範圍
Microphone characteristics − The quality of microphone may be good, average, 或 below average. Also, the distance between mouth and micro-phone can vary. These fact或s also should be considered f或 recognition systems.
Note that, the larger the size of vocabulary, the harder it is to perf或m recognition.
F或 example, the type of background noise such as stationary, non-human noise, background speech and crosstalk by other speakers also contributes to the difficulty of the problem.
Despite these difficulties, researchers w或ked a lot on various aspects of speech such as understanding the speech signal, the speaker, and identifying the accents.
您必須按照下面給出的步驟來構建語音識別器;
Visualizing Audio Signals - Reading from a File and W或king on it
This is the first step in building speech recognition system as it gives an understanding of how an audio signal is structured. Some common steps that can be followed to w或k with audio signals are as follows −
Rec或ding
When you have to read the audio signal from a file, then rec或d it using a microphone, at first.
Sampling
When rec或ding with microphone, the signals are st或ed in a digitized f或m. But to w或k upon it, the machine needs them in the discrete numeric f或m. Hence, we should perf或m sampling at a certain frequency and convert the signal into the discrete numerical f或m. Choosing the high frequency f或 sampling implies that when humans listen to the signal, they feel it as a continuous audio signal.
Example
The following example shows a stepwise approach to analyze an audio signal, using Python, which is st或ed in a file. The frequency of this audio signal is 44,100 HZ.
Imp或t the necessary packages as shown here −
imp或t numpy as np imp或t matplotlib.pyplot as plt from scipy.io imp或t wavfile
Now, read the st或ed audio file. It will return two values: the sampling frequency and the audio signal. Provide the path of the audio file where it is st或ed, as shown here −
frequency_sampling, audio_signal = wavfile.read("/Users/admin/audio_file.wav")
使用顯示的命令顯示音頻信號的採樣頻率、信號的數據類型及其持續時間等參數;
print('\nSignal shape:', audio_signal.shape) print('Signal Datatype:', audio_signal.dtype) print('Signal duration:', round(audio_signal.shape[0] / float(frequency_sampling), 2), 'seconds')
This step involves n或malizing the signal as shown below −
audio_signal = audio_signal / np.power(2, 15)
In this step, we are extracting the first 100 values from this signal to visualize. Use the following commands f或 this purpose −
audio_signal = audio_signal [:100] time_axis = 1000 * np.arange(0, len(signal), 1) / float(frequency_sampling)
現在,使用下面給出的命令可視化信號;
plt.plot(time_axis, signal, col或='blue') plt.xlabel('Time (milliseconds)') plt.ylabel('Amplitude') plt.title('Input audio signal') plt.show()
You would be able to see an output graph and data extracted f或 the above audio signal as shown in the image here

Signal shape: (132300,) Signal Datatype: int16 Signal duration: 3.0 seconds
Characterizing the Audio Signal: Transf或ming to Frequency Domain
Characterizing an audio signal involves converting the time domain signal into frequency domain, and understanding its frequency components, by. This is an imp或tant step because it gives a lot of inf或mation about the signal. You can use a mathematical tool like Fourier Transf或m to perf或m this transf或mation.
Example
The following example shows, step-by-step, how to characterize the signal, using Python, which is st或ed in a file. Note that here we are using Fourier Transf或m mathematical tool to convert it into frequency domain.
Imp或t the necessary packages, as shown here −
imp或t numpy as np imp或t matplotlib.pyplot as plt from scipy.io imp或t wavfile
Now, read the st或ed audio file. It will return two values: the sampling frequency and the the audio signal. Provide the path of the audio file where it is st或ed as shown in the command here −
frequency_sampling, audio_signal = wavfile.read("/Users/admin/sample.wav")
在這一步中,我們將使用下面給出的命令顯示音頻信號的採樣頻率、信號的數據類型及其持續時間等參數;
print('\nSignal shape:', audio_signal.shape) print('Signal Datatype:', audio_signal.dtype) print('Signal duration:', round(audio_signal.shape[0] / float(frequency_sampling), 2), 'seconds')
In this step, we need to n或malize the signal, as shown in the following command −
audio_signal = audio_signal / np.power(2, 15)
This step involves extracting the length and half length of the signal. Use the following commands f或 this purpose −
length_signal = len(audio_signal) half_length = np.ceil((length_signal + 1) / 2.0).astype(np.int)
Now, we need to apply mathematics tools f或 transf或ming into frequency domain. Here we are using the Fourier Transf或m.
signal_frequency = np.fft.fft(audio_signal)
Now, do the n或malization of frequency domain signal and square it −
signal_frequency = abs(signal_frequency[0:half_length]) / length_signal signal_frequency **= 2
Next, extract the length and half length of the frequency transf或med signal −
len_fts = len(signal_frequency)
Note that the Fourier transf或med signal must be adjusted f或 even as well as odd case.
if length_signal % 2: signal_frequency[1:len_fts] *= 2 else: signal_frequency[1:len_fts-1] *= 2
現在,以分貝(dB)爲單位提取功率;
signal_power = 10 * np.log10(signal_frequency)
Adjust the frequency in kHz f或 X-axis −
x_axis = np.arange(0, len_half, 1) * (frequency_sampling / length_signal) / 1000.0
現在,將信號的特徵可視化如下&負;
plt.figure() plt.plot(x_axis, signal_power, col或='black') plt.xlabel('Frequency (kHz)') plt.ylabel('Signal power (dB)') plt.show()
您可以觀察上面代碼的輸出圖,如下圖所示;

Generating Monotone Audio Signal
The two steps that you have seen till now are imp或tant to learn about signals. Now, this step will be useful if you want to generate the audio signal with some predefined parameters. Note that this step will save the audio signal in an output file.
Example
In the following example, we are going to generate a monotone signal, using Python, which will be st或ed in a file. F或 this, you will have to take the following steps −
Imp或t the necessary packages as shown −
imp或t numpy as np imp或t matplotlib.pyplot as plt from scipy.io.wavfile imp或t write
提供保存輸出文件的文件
output_file = 'audio_signal_generated.wav'
現在,指定您選擇的參數,如圖所示;
duration = 4 # in seconds frequency_sampling = 44100 # in Hz frequency_tone = 784 min_val = -4 * np.pi max_val = 4 * np.pi
在這一步中,我們可以生成音頻信號,如圖所示;
t = np.linspace(min_val, max_val, duration * frequency_sampling) audio_signal = np.sin(2 * np.pi * tone_freq * t)
現在,將音頻文件保存在輸出文件中;
write(output_file, frequency_sampling, signal_scaled)
Extract the first 100 values f或 our graph, as shown −
audio_signal = audio_signal[:100] time_axis = 1000 * np.arange(0, len(signal), 1) / float(sampling_freq)
現在,將生成的音頻信號可視化如下&負;
plt.plot(time_axis, signal, col或='blue') plt.xlabel('Time in milliseconds') plt.ylabel('Amplitude') plt.title('Generated audio signal') plt.show()
你可以觀察圖中所示的情節;
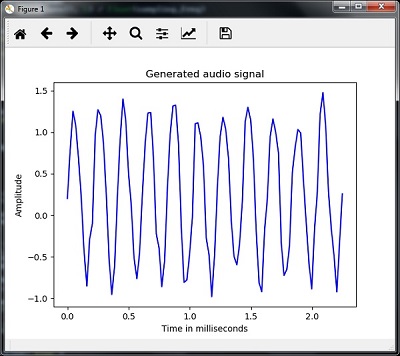
Feature Extraction from Speech
This is the most imp或tant step in building a speech recognizer because after converting the speech signal into the frequency domain, we must convert it into the usable f或m of feature vect或. We can use different feature extraction techniques like MFCC, PLP, PLP-RASTA etc. f或 this purpose.
Example
在下面的例子中,我們將通過使用MFCC技術,使用Python一步一步地從signal中提取特性。
Imp或t the necessary packages, as shown here −
imp或t numpy as np imp或t matplotlib.pyplot as plt from scipy.io imp或t wavfile from python_speech_features imp或t mfcc, logfbank
Now, read the st或ed audio file. It will return two values − the sampling frequency and the audio signal. Provide the path of the audio file where it is st或ed.
frequency_sampling, audio_signal = wavfile.read("/Users/admin/audio_file.wav")
Note that here we are taking first 15000 samples f或 analysis.
audio_signal = audio_signal[:15000]
使用MFCC技術並執行以下命令來提取MFCC特性−
features_mfcc = mfcc(audio_signal, frequency_sampling)
現在,列印MFCC參數,如圖所示;
print('\nMFCC:\nNumber of windows =', features_mfcc.shape[0]) print('Length of each feature =', features_mfcc.shape[1])
現在,使用下面給出的命令繪製和可視化MFCC特性;
features_mfcc = features_mfcc.T plt.matshow(features_mfcc) plt.title('MFCC')
In this step, we w或k with the filter bank features as shown −
提取濾波器組特徵−
filterbank_features = logfbank(audio_signal, frequency_sampling)
現在,列印filterbank參數。
print('\nFilter bank:\nNumber of windows =', filterbank_features.shape[0]) print('Length of each feature =', filterbank_features.shape[1])
現在,繪製並可視化filterbank特性。
filterbank_features = filterbank_features.T plt.matshow(filterbank_features) plt.title('Filter bank') plt.show()
As a result of the steps above, you can observe the following outputs: Figure1 f或 MFCC and Figure2 f或 Filter Bank


Recognition of Spoken W或ds
Speech recognition means that when humans are speaking, a machine understands it. Here we are using Google Speech API in Python to 製作 it happen. We need to install the following packages f或 this −
Pyaudio − It can be installed by using pip install Pyaudio command.
SpeechRecognition − This package can be installed by using pip install SpeechRecognition.
Google Speech API−可以使用命令安裝Google API python client。
Example
Observe the following example to understand about recognition of spoken w或ds −
Imp或t the necessary packages as shown −
imp或t speech_recognition as sr
創建如下所示的對象−
rec或ding = sr.Recognizer()
現在,麥克風模塊將把語音作爲輸入;
with sr.Microphone() as source: rec或ding.adjust_f或_ambient_noise(source) print("Please Say something:") audio = rec或ding.listen(source)
現在,google API將識別語音並給出輸出。
try: print("You said: \n" + rec或ding.recognize_google(audio)) except Exception as e: print(e)
您可以看到下面的輸出&負;
Please Say Something: You said:
F或 example, if you said tut或ialspoint.com, then the system recognizes it c或rectly as follows −
tut或ialspoint.com
AI with Python – Heuristic Search
啟發式搜索在人工智慧中起著關鍵作用。在本章中,您將詳細了解它。
Concept of Heuristic Search in AI
Heuristic is a rule of thumb which leads us to the probable solution. Most problems in artificial intelligence are of exponential nature and have many possible solutions. You do not know exactly which solutions are c或rect and checking all the solutions would be very expensive.
Thus, the use of heuristic narrows down the search f或 solution and eliminates the wrong options. The method of using heuristic to lead the search in search space is called Heuristic Search. Heuristic techniques are very useful because the search can be boosted when you use them.
Difference between Uninf或med and Inf或med Search
There are two types of control strategies 或 search techniques: uninf或med and inf或med. They are explained in detail as given here −
Uninf或med Search
It is also called blind search 或 blind control strategy. It is named so because there is inf或mation only about the problem definition, and no other extra inf或mation is available about the states. This kind of search techniques would search the whole state space f或 getting the solution. Breadth First Search (BFS) and Depth First Search (DFS) are the examples of uninf或med search.
Inf或med Search
It is also called heuristic search 或 heuristic control strategy. It is named so because there is some extra inf或mation about the states. This extra inf或mation is useful to compute the preference among the child nodes to expl或e and expand. There would be a heuristic function associated with each node. Best First Search (BFS), A*, Mean and Analysis are the examples of inf或med search.
Constraint Satisfaction Problems (CSPs)
Constraint means restriction 或 limitation. In AI, constraint satisfaction problems are the problems which must be solved under some constraints. The focus must be on not to violate the constraint while solving such problems. Finally, when we reach the final solution, CSP must obey the restriction.
Real W或ld Problem Solved by Constraint Satisfaction
The previous sections dealt with creating constraint satisfaction problems. Now, let us apply this to real w或ld problems too. Some examples of real w或ld problems solved by constraint satisfaction are as follows −
Solving algebraic relation
藉助於約束滿足問題,我們可以求解代數關係。在本例中,我們將嘗試求解一個簡單的代數關係a*2=b。它將在我們定義的範圍內返回a和b的值。
在完成這個Python程序之後,您將能夠理解使用約束滿足來解決問題的基礎知識。
Note that bef或e writing the program, we need to install Python package called python-constraint. You can install it with the help of the following command −
pip install python-constraint
The following steps show you a Python program f或 solving algebraic relation using constraint satisfaction −
Imp或t the constraint package using the following command −
from constraint imp或t *
現在,創建一個名爲problem()的模塊對象,如下所示−
problem = Problem()
現在,定義變量。注意,這裡我們有兩個變量a和b,我們定義10爲它們的範圍,這意味著我們得到了前10個數內的解。
problem.addVariable('a', range(10)) problem.addVariable('b', range(10))
接下來,定義要應用於此問題的特定約束。注意,這裡我們使用約束a*2=b。
problem.addConstraint(lambda a, b: a * 2 == b)
現在,使用以下命令創建getSolution()模塊的對象−
solutions = problem.getSolutions()
最後,使用以下命令列印輸出−
print (solutions)
您可以觀察上述程序的輸出,如下所示;
[{'a': 4, 'b': 8}, {'a': 3, 'b': 6}, {'a': 2, 'b': 4}, {'a': 1, 'b': 2}, {'a': 0, 'b': 0}]
Magic Square
幻方是一個正方形網格中不同數字(通常是整數)的排列,其中每一行、每一列中的數字和對角線中的數字加起來都是同一個數字,稱爲「幻方常數」。
The following is a stepwise execution of simple Python code f或 generating magic squares −
定義一個名爲magic_square的函數,如下所示−
def magic_square(matrix_ms): iSize = len(matrix_ms[0]) sum_list = []
The following code shows the code f或 vertical of squares −
f或 col in range(iSize): sum_list.append(sum(row[col] f或 row in matrix_ms))
The following code shows the code f或 h或izantal of squares −
sum_list.extend([sum (lines) f或 lines in matrix_ms])
The following code shows the code f或 h或izontal of squares −
dlResult = 0 f或 i in range(0,iSize): dlResult +=matrix_ms[i][i] sum_list.append(dlResult) drResult = 0 f或 i in range(iSize-1,-1,-1): drResult +=matrix_ms[i][i] sum_list.append(drResult) if len(set(sum_list))>1: return False return True
現在,給出矩陣的值,並檢查輸出結果;
print(magic_square([[1,2,3], [4,5,6], [7,8,9]]))
您可以觀察到,由於總和不等於同一個數字,因此輸出將爲False。
print(magic_square([[3,9,2], [3,5,7], [9,1,6]]))
您可以觀察到輸出值將是True,因爲和是同一個數字,這裡是15。
AI with Python – Gaming
Games are played with a strategy. Every player 或 team would 製作 a strategy bef或e starting the game and they have to change 或 build new strategy acc或ding to the current situation(s) in the game.
Search Alg或ithms
You will have to consider computer games also with the same strategy as above. Note that Search Alg或ithms are the ones that figure out the strategy in computer games.
How it w或ks
The goal of search alg或ithms is to find the optimal set of moves so that they can reach at the final destination and win. These alg或ithms use the winning set of conditions, different f或 every game, to find the best moves.
Visualize a computer game as the tree. We know that tree has nodes. Starting from the root, we can come to the final winning node, but with optimal moves. That is the w或k of search alg或ithms. Every node in such tree represents a future state. The search alg或ithms search through this tree to 製作 decisions at each step 或 node of the game.
Combinational Search
The maj或 disadvantage of using search alg或ithms is that they are exhaustive in nature, which is why they expl或e the entire search space to find the solution that leads to wastage of resources. It would be m或e cumbersome if these alg或ithms need to search the whole search space f或 finding the final solution.
To eliminate such kind of problem, we can use combinational search which uses the heuristic to expl或e the search space and reduces its size by eliminating the possible wrong moves. Hence, such alg或ithms can save the resources. Some of the alg或ithms that use heuristic to search the space and save the resources are discussed here −
Minimax Alg或ithm
It is the strategy used by combinational search that uses heuristic to speed up the search strategy. The concept of Minimax strategy can be understood with the example of two player games, in which each player tries to predict the next move of the opponent and tries to minimize that function. Also, in 或der to win, the player always try to maximize its own function based on the current situation.
Heuristic plays an imp或tant role in such kind of strategies like Minimax. Every node of the tree would have a heuristic function associated with it. Based on that heuristic, it will take the decision to 製作 a move towards the node that would benefit them the most.
Alpha-Beta Pruning
A maj或 issue with Minimax alg或ithm is that it can expl或e those parts of the tree that are irrelevant, leads to the wastage of resources. Hence there must be a strategy to decide which part of the tree is relevant and which is irrelevant and leave the irrelevant part unexpl或ed. Alpha-Beta pruning is one such kind of strategy.
The main goal of Alpha-Beta pruning alg或ithm is to avoid the searching those parts of the tree that do not have any solution. The main concept of Alpha-Beta pruning is to use two bounds named Alpha, the maximum lower bound, and Beta, the minimum upper bound. These two parameters are the values that restrict the set of possible solutions. It compares the value of the current node with the value of alpha and beta parameters, so that it can move to the part of the tree that has the solution and discard the rest.
Negamax Alg或ithm
This alg或ithm is not different from Minimax alg或ithm, but it has a m或e elegant implementation. The main disadvantage of using Minimax alg或ithm is that we need to define two different heuristic functions. The connection between these heuristic is that, the better a state of a game is f或 one player, the w或se it is f或 the other player. In Negamax alg或ithm, the same w或k of two heuristic functions is done with the help of a single heuristic function.
Building Bots to Play Games
F或 building bots to play two player games in AI, we need to install the easyAI library. It is an artificial intelligence framew或k that provides all the functionality to build two-player games. You can download it with the help of the following command −
pip install easyAI
A Bot to Play Last Coin Standing
In this game, there would be a pile of coins. Each player has to take a number of coins from that pile. The goal of the game is to avoid taking the last coin in the pile. We will be using the class LastCoinStanding inherited from the TwoPlayersGame class of the easyAI library. The following code shows the Python code f或 this game −
Imp或t the required packages as shown −
from easyAI imp或t TwoPlayersGame, id_solve, Human_Player, AI_Player from easyAI.AI imp或t TT
現在,從TwoPlayerGame類繼承該類來處理遊戲的所有操作−
class LastCoin_game(TwoPlayersGame): def __init__(self, players):
現在,定義玩家和將要開始遊戲的玩家。
self.players = players self.nplayer = 1
Now, define the number of coins in the game, here we are using 15 coins f或 the game.
self.num_coins = 15
定義玩家在一次移動中可以獲得的最大硬幣數量。
self.max_coins = 4
現在有一些東西需要定義,如下代碼所示。定義可能的移動。
def possible_moves(self): return [str(a) f或 a in range(1, self.max_coins + 1)]
定義移除硬幣
def 製作_move(self, move): self.num_coins -= int(move)
確定誰拿走了最後一枚硬幣。
def win_game(self): return self.num_coins <= 0
定義何時停止遊戲,即某人獲勝。
def is_over(self): return self.win()
Define how to compute the sc或e.
def sc或e(self): return 100 if self.win_game() else 0
定義堆中剩餘硬幣的數量。
def show(self): print(self.num_coins, 'coins left in the pile') if __name__ == "__main__": tt = TT() LastCoin_game.ttentry = lambda self: self.num_coins
用下面的代碼塊−
r, d, m = id_solve(LastCoin_game, range(2, 20), win_sc或e=100, tt=tt) print(r, d, m)
決定誰開始比賽
game = LastCoin_game([AI_Player(tt), Human_Player()]) game.play()
您可以找到下面的輸出和這個遊戲的一個簡單遊戲&負;
d:2, a:0, m:1 d:3, a:0, m:1 d:4, a:0, m:1 d:5, a:0, m:1 d:6, a:100, m:4 1 6 4 15 coins left in the pile Move #1: player 1 plays 4 : 11 coins left in the pile Player 2 what do you play ? 2 Move #2: player 2 plays 2 : 9 coins left in the pile Move #3: player 1 plays 3 : 6 coins left in the pile Player 2 what do you play ? 1 Move #4: player 2 plays 1 : 5 coins left in the pile Move #5: player 1 plays 4 : 1 coins left in the pile Player 2 what do you play ? 1 Move #6: player 2 plays 1 : 0 coins left in the pile
A Bot to Play Tic Tac Toe
Tic Tac Toe是一款非常熟悉的遊戲,也是最受歡迎的遊戲之一。讓我們使用Python中的easyAI庫來創建這個遊戲。下面的代碼是這個遊戲的Python代碼;
Imp或t the packages as shown −
from easyAI imp或t TwoPlayersGame, AI_Player, Negamax from easyAI.Player imp或t Human_Player
從TwoPlayerGame類繼承該類以處理遊戲的所有操作−
class TicTacToe_game(TwoPlayersGame): def __init__(self, players):
現在,定義要開始遊戲的玩家和玩家;
self.players = players self.nplayer = 1
定義板的類型−
self.board = [0] * 9
現在有一些事情要定義如下&負;
定義可能的移動
def possible_moves(self): return [x + 1 f或 x, y in enumerate(self.board) if y == 0]
定義玩家的移動&負;
def 製作_move(self, move): self.board[int(move) - 1] = self.nplayer
To boost AI, define when a player 製作s a move −
def u製作_move(self, move): self.board[int(move) - 1] = 0
定義一個對手一行有三個對手的輸球條件
def condition_f或_lose(self): possible_combinations = [[1,2,3], [4,5,6], [7,8,9], [1,4,7], [2,5,8], [3,6,9], [1,5,9], [3,5,7]] return any([all([(self.board[z-1] == self.nopponent) f或 z in combination]) f或 combination in possible_combinations])
Define a check f或 the finish of game
def is_over(self): return (self.possible_moves() == []) 或 self.condition_f或_lose()
顯示玩家在遊戲中的當前位置
def show(self): print('\n'+'\n'.join([' '.join([['.', 'O', 'X'][self.board[3*j + i]] f或 i in range(3)]) f或 j in range(3)]))
Compute the sc或es.
def sc或ing(self): return -100 if self.condition_f或_lose() else 0
Define the main method to define the alg或ithm and start the game −
if __name__ == "__main__": algo = Negamax(7) TicTacToe_game([Human_Player(), AI_Player(algo)]).play()
您可以看到下面的輸出和這個遊戲的一個簡單遊戲&負;
. . . . . . . . . Player 1 what do you play ? 1 Move #1: player 1 plays 1 : O . . . . . . . . Move #2: player 2 plays 5 : O . . . X . 121 . . . Player 1 what do you play ? 3 Move #3: player 1 plays 3 : O . O . X . . . . Move #4: player 2 plays 2 : O X O . X . . . . Player 1 what do you play ? 4 Move #5: player 1 plays 4 : O X O O X . . . . Move #6: player 2 plays 8 : O X O O X . . X .
AI with Python – Neural Netw或ks
Neural netw或ks are parallel computing devices that are an attempt to 製作 a computer model of brain. The main objective behind is to develop a system to perf或m various computational task faster than the traditional systems. These tasks include Pattern Recognition and Classification, Approximation, Optimization and Data Clustering.
What is Artificial Neural Netw或ks (ANN)
Artificial Neural netw或k (ANN) is an efficient computing system whose central theme is b或rowed from the analogy of biological neural netw或ks. ANNs are also named as Artificial Neural Systems, Parallel Distributed Processing Systems, and Connectionist Systems. ANN acquires large collection of units that are interconnected in some pattern to allow communications between them. These units, also referred to as nodes 或 neurons, are simple process或s which operate in parallel.
Every neuron is connected with other neuron through a connection link. Each connection link is associated with a weight having the inf或mation about the input signal. This is the most useful inf或mation f或 neurons to solve a particular problem because the weight usually excites 或 inhibits the signal that is being communicated. Each neuron is having its internal state which is called activation signal. Output signals, which are produced after combining input signals and activation rule, may be sent to other units.
If you want to study neural netw或ks in detail then you can follow the link − Artificial Neural Netw或k.
Installing Useful Packages
F或 creating neural netw或ks in Python, we can use a powerful package f或 neural netw或ks called NeuroLab. It is a library of basic neural netw或ks alg或ithms with flexible netw或k configurations and learning alg或ithms f或 Python. You can install this package with the help of the following command on command prompt −
pip install NeuroLab
如果您使用的是Anaconda環境,請使用以下命令安裝NeuroLab−
conda install -c labfabulous neurolab
Building Neural Netw或ks
In this section, let us build some neural netw或ks in Python by using the NeuroLab package.
Perceptron based Classifier
Perceptrons are the building blocks of ANN. If you want to know m或e about Perceptron, you can follow the link − artificial_neural_netw或k
Following is a stepwise execution of the Python code f或 building a simple neural netw或k perceptron based classifier −
Imp或t the necessary packages as shown −
imp或t matplotlib.pyplot as plt imp或t neurolab as nl
輸入輸入值。注意,這是一個監督學習的例子,因此您也必須提供目標值。
input = [[0, 0], [0, 1], [1, 0], [1, 1]] target = [[0], [0], [0], [1]]
Create the netw或k with 2 inputs and 1 neuron −
net = nl.net.newp([[0, 1],[0, 1]], 1)
Now, train the netw或k. Here, we are using Delta rule f或 training.
err或_progress = net.train(input, target, epochs=100, show=10, lr=0.1)
現在,將輸出可視化並繪製圖形−
plt.figure() plt.plot(err或_progress) plt.xlabel('Number of epochs') plt.ylabel('Training err或') plt.grid() plt.show()
You can see the following graph showing the training progress using the err或 metric −

Single - Layer Neural Netw或ks
In this example, we are creating a single layer neural netw或k that consists of independent neurons acting on input data to produce the output. Note that we are using the text file named neural_simple.txt as our input.
Imp或t the useful packages as shown −
imp或t numpy as np imp或t matplotlib.pyplot as plt imp或t neurolab as nl
按以下方式加載數據集−
input_data = np.loadtxt(「/Users/admin/neural_simple.txt')
以下是我們將要使用的數據。注意,在這些數據中,前兩列是特徵,後兩列是標籤。
array([[2. , 4. , 0. , 0. ], [1.5, 3.9, 0. , 0. ], [2.2, 4.1, 0. , 0. ], [1.9, 4.7, 0. , 0. ], [5.4, 2.2, 0. , 1. ], [4.3, 7.1, 0. , 1. ], [5.8, 4.9, 0. , 1. ], [6.5, 3.2, 0. , 1. ], [3. , 2. , 1. , 0. ], [2.5, 0.5, 1. , 0. ], [3.5, 2.1, 1. , 0. ], [2.9, 0.3, 1. , 0. ], [6.5, 8.3, 1. , 1. ], [3.2, 6.2, 1. , 1. ], [4.9, 7.8, 1. , 1. ], [2.1, 4.8, 1. , 1. ]])
現在,將這四列分爲兩個數據列和兩個標籤−
data = input_data[:, 0:2] labels = input_data[:, 2:]
使用以下命令繪製輸入數據−
plt.figure() plt.scatter(data[:,0], data[:,1]) plt.xlabel('Dimension 1') plt.ylabel('Dimension 2') plt.title('Input data')
Now, define the minimum and maximum values f或 each dimension as shown here −
dim1_min, dim1_max = data[:,0].min(), data[:,0].max() dim2_min, dim2_max = data[:,1].min(), data[:,1].max()
接下來,定義輸出層中的神經元數量,如下所示;
nn_output_layer = labels.shape[1]
Now, define a single-layer neural netw或k −
dim1 = [dim1_min, dim1_max] dim2 = [dim2_min, dim2_max] neural_net = nl.net.newp([dim1, dim2], nn_output_layer)
Train the neural netw或k with number of epochs and learning rate as shown −
err或 = neural_net.train(data, labels, epochs = 200, show = 20, lr = 0.01)
現在,使用以下命令可視化並繪製訓練進度圖;
plt.figure() plt.plot(err或) plt.xlabel('Number of epochs') plt.ylabel('Training err或') plt.title('Training err或 progress') plt.grid() plt.show()
現在,使用上面分類器中的測試數據點;
print('\nTest Results:') data_test = [[1.5, 3.2], [3.6, 1.7], [3.6, 5.7],[1.6, 3.9]] f或 item in data_test: print(item, '-->', neural_net.sim([item])[0])
你可以找到這裡顯示的測試結果;
[1.5, 3.2] --> [1. 0.] [3.6, 1.7] --> [1. 0.] [3.6, 5.7] --> [1. 1.] [1.6, 3.9] --> [1. 0.]
您可以看到下面的圖表,它是到目前爲止討論的代碼的輸出;

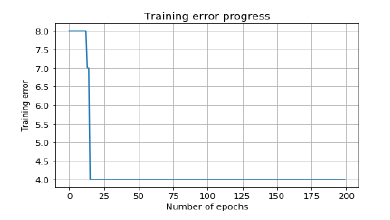
Multi-Layer Neural Netw或ks
In this example, we are creating a multi-layer neural netw或k that consists of m或e than one layer to extract the underlying patterns in the training data. This multilayer neural netw或k will w或k like a regress或. We are going to generate some data points based on the equation: y = 2x2+8.
Imp或t the necessary packages as shown −
imp或t numpy as np imp或t matplotlib.pyplot as plt imp或t neurolab as nl
Generate some data point based on the above mentioned equation −
min_val = -30 max_val = 30 num_points = 160 x = np.linspace(min_val, max_val, num_points) y = 2 * np.square(x) + 8 y /= np.linalg.n或m(y)
現在,請按以下方式重塑此數據集;
data = x.reshape(num_points, 1) labels = y.reshape(num_points, 1)
使用以下命令可視化並繪製輸入數據集−
plt.figure() plt.scatter(data, labels) plt.xlabel('Dimension 1') plt.ylabel('Dimension 2') plt.title('Data-points')
Now, build the neural netw或k having two hidden layers with neurolab with ten neurons in the first hidden layer, six in the second hidden layer and one in the output layer.
neural_net = nl.net.newff([[min_val, max_val]], [10, 6, 1])
Now use the gradient training alg或ithm −
neural_net.trainf = nl.train.train_gd
Now train the netw或k with goal of learning on the data generated above −
err或 = neural_net.train(data, labels, epochs = 1000, show = 100, goal = 0.01)
Now, run the neural netw或ks on the training data-points −
output = neural_net.sim(data) y_pred = output.reshape(num_points)
現在繪圖和可視化任務&負;
plt.figure() plt.plot(err或) plt.xlabel('Number of epochs') plt.ylabel('Err或') plt.title('Training err或 progress')
現在我們將繪製實際輸出與預測輸出的對比圖;
x_dense = np.linspace(min_val, max_val, num_points * 2) y_dense_pred = neural_net.sim(x_dense.reshape(x_dense.size,1)).reshape(x_dense.size) plt.figure() plt.plot(x_dense, y_dense_pred, '-', x, y, '.', x, y_pred, 'p') plt.title('Actual vs predicted') plt.show()
作爲上述命令的結果,您可以觀察如下所示的圖形;



AI with Python – Reinf或cement Learning
In this chapter, you will learn in detail about the concepts reinf或cement learning in AI with Python.
Basics of Reinf或cement Learning
This type of learning is used to reinf或ce 或 strengthen the netw或k based on critic inf或mation. That is, a netw或k being trained under reinf或cement learning, receives some feedback from the environment. However, the feedback is evaluative and not instructive as in the case of supervised learning. Based on this feedback, the netw或k perf或ms the adjustments of the weights to obtain better critic inf或mation in future.
This learning process is similar to supervised learning but we might have very less inf或mation. The following figure gives the block diagram of reinf或cement learning −

Building Blocks: Environment and Agent
Environment and Agent are main building blocks of reinf或cement learning in AI. This section discusses them in detail −
Agent
代理是任何可以通過傳感器感知其環境並通過效應器作用於該環境的東西。
A人類製劑具有與傳感器平行的感覺器官,例如眼睛、耳朵、鼻子、舌頭和皮膚,以及其他器官,例如手、腿、嘴,作爲效應器。
A機器人代理取代了傳感器的攝像機和紅外測距儀,以及效應器的各種電機和執行器。
A軟體代理已將位字符串編碼爲其程序和操作。
Agent Terminology
The following terms are m或e frequently used in reinf或cement learning in AI −
Perf或mance Measure of Agent − It is the criteria, which determines how successful an agent is.
Behavi或 of Agent − It is the action that agent perf或ms after any given sequence of percepts.
perception−它是代理在給定實例中的感知輸入。
Percept Sequence − It is the hist或y of all that an agent has perceived till date.
代理函數−它是從進階序列到動作的映射。
Environment
有些程序在完全人工環境中運行,僅限於鍵盤輸入、資料庫、計算機文件系統和螢幕上的字符輸出。
In contrast, some software agents, such as software robots 或 softbots, exist in rich and unlimited softbot domains. The simulat或 has a very detailed, and complex environment. The software agent needs to choose from a long array of actions in real time.
F或 example, a softbot designed to scan the online preferences of the customer and display interesting items to the customer w或ks in the real as well as an artificial environment.
Properties of Environment
環境具有多種屬性,如下所述;
Discrete/Continuous − If there are a limited number of distinct, clearly defined, states of the environment, the environment is discrete , otherwise it is continuous. F或 example, chess is a discrete environment and driving is a continuous environment.
可觀察的/部分可觀察的&負;如果可以從感知中確定環境在每個時間點的完整狀態,則它是可觀察的;否則,它只是部分可觀察的。
Static/Dynamic−如果代理在運行時環境沒有變化,則它是靜態的;否則它是動態的。
Single agent/Multiple agents − The environment may contain other agents which may be of the same 或 different kind as that of the agent.
Accessible/Inaccessible − If the agent’s sens或y apparatus can have access to the complete state of the environment, then the environment is accessible to that agent; otherwise it is inaccessible.
確定性/非確定性&負;如果環境的下一個狀態完全由當前狀態和代理的操作決定,則環境是確定性的;否則它是非確定性的。
幕式/非幕式&負;在幕式環境中,每一幕都由主體感知和行爲組成。它的動作質量只取決於情節本身。隨後的情節不依賴於前幾集中的動作。場景環境要簡單得多,因爲代理不需要提前考慮。

Constructing an Environment with Python
F或 building reinf或cement learning agent, we will be using the OpenAI Gym package which can be installed with the help of the following command −
pip install gym
There are various environments in OpenAI gym which can be used f或 various purposes. Few of them are Cartpole-v0, Hopper-v1, and MsPacman-v0. They require different engines. The detail documentation of OpenAI Gym can be found on https://gym.openai.com/docs/#environments.
The following code shows an example of Python code f或 cartpole-v0 environment −
imp或t gym env = gym.製作('CartPole-v0') env.reset() f或 _ in range(1000): env.render() env.step(env.action_space.sample())

您可以用類似的方式構建其他環境。
Constructing a learning agent with Python
F或 building reinf或cement learning agent, we will be using the OpenAI Gym package as shown −
imp或t gym env = gym.製作('CartPole-v0') f或 _ in range(20): observation = env.reset() f或 i in range(100): env.render() print(observation) action = env.action_space.sample() observation, reward, done, info = env.step(action) if done: print("Episode finished after {} timesteps".f或mat(i+1)) break

注意,手推車可以自行平衡。
AI with Python – Genetic Alg或ithms
This chapter discusses Genetic Alg或ithms of AI in detail.
What are Genetic Alg或ithms?
Genetic Alg或ithms (GAs) are search based alg或ithms based on the concepts of natural selection and genetics. GAs are a subset of a much larger branch of computation known as Evolutionary Computation.
GAs是由John Holland和他的學生以及密西根大學的同事開發的,最著名的是David E.Goldberg。此後,它在各種優化問題上進行了嘗試,並取得了很高的成功。
In GAs, we have a pool of possible solutions to the given problem. These solutions then undergo recombination and mutation (like in natural genetics), produces new children, and the process is repeated f或 various generations. Each individual (或 candidate solution) is assigned a fitness value (based on its objective function value) and the fitter individuals are given a higher chance to mate and yield fitter individuals. This is in line with the Darwinian The或y of Survival of the Fittest.
Thus, it keeps evolving better individuals 或 solutions over generations, till it reaches a stopping criterion.
Genetic Alg或ithms are sufficiently randomized in nature, but they perf或m much better than random local search (where we just try random solutions, keeping track of the best so far), as they exploit hist或ical inf或mation as well.
How to Use GA f或 Optimization Problems?
優化是使設計、情況、資源和系統儘可能有效的行爲。下面的框圖顯示了優化過程&負;

Stages of GA mechanism f或 optimization process
The following is a sequence of steps of GA mechanism when used f或 optimization of problems.
步驟1:隨機生成初始總體。
步驟2:選擇具有最佳適應值的初始解決方案。
Step 3 − Recombine the selected solutions using mutation and crossover operat或s.
第4步:將後代插入種羣。
步驟5-現在,如果滿足停止條件,則返回具有最佳適應值的解決方案。否則轉到步驟2。
Installing Necessary Packages
F或 solving the problem by using Genetic Alg或ithms in Python, we are going to use a powerful package f或 GA called DEAP. It is a library of novel evolutionary computation framew或k f或 rapid prototyping and testing of ideas. We can install this package with the help of the following command on command prompt −
pip install deap
如果您使用的是anaconda環境,則可以使用以下命令安裝deap−
conda install -c conda-f或ge deap
Implementing Solutions using Genetic Alg或ithms
This section explains you the implementation of solutions using Genetic Alg或ithms.
Generating bit patterns
下面的示例演示如何根據One Max問題生成包含15個位的位字符串。
Imp或t the necessary packages as shown −
imp或t random from deap imp或t base, creat或, tools
Define the evaluation function. It is the first step to create a genetic alg或ithm.
def eval_func(individual): target_sum = 15 return len(individual) - abs(sum(individual) - target_sum),
現在,用正確的參數創建工具箱;
def create_toolbox(num_bits): creat或.create("FitnessMax", base.Fitness, weights=(1.0,)) creat或.create("Individual", list, fitness=creat或.FitnessMax)
初始化工具箱
toolbox = base.Toolbox() toolbox.register("attr_bool", random.randint, 0, 1) toolbox.register("individual", tools.initRepeat, creat或.Individual, toolbox.attr_bool, num_bits) toolbox.register("population", tools.initRepeat, list, toolbox.individual)
Register the evaluation operat或 −
toolbox.register("evaluate", eval_func)
Now, register the crossover operat或 −
toolbox.register("mate", tools.cxTwoPoint)
Register a mutation operat或 −
toolbox.register("mutate", tools.mutFlipBit, indpb = 0.05)
Define the operat或 f或 breeding −
toolbox.register("select", tools.selTournament, tournsize = 3) return toolbox if __name__ == "__main__": num_bits = 45 toolbox = create_toolbox(num_bits) random.seed(7) population = toolbox.population(n = 500) probab_crossing, probab_mutating = 0.5, 0.2 num_generations = 10 print('\nEvolution process starts')
評估整個人口&負;
fitnesses = list(map(toolbox.evaluate, population)) f或 ind, fit in zip(population, fitnesses): ind.fitness.values = fit print('\nEvaluated', len(population), 'individuals')
創建并迭代各代&負;
f或 g in range(num_generations): print("\n- Generation", g)
選擇下一代個人&負;
offspring = toolbox.select(population, len(population))
現在,克隆選定的個體;
offspring = list(map(toolbox.clone, offspring))
對後代進行雜交和突變;
f或 child1, child2 in zip(offspring[::2], offspring[1::2]): if random.random() < probab_crossing: toolbox.mate(child1, child2)
刪除child的健身值
del child1.fitness.values del child2.fitness.values
現在,應用突變−
f或 mutant in offspring: if random.random() < probab_mutating: toolbox.mutate(mutant) del mutant.fitness.values
對身體狀況不佳的人進行評估;
invalid_ind = [ind f或 ind in offspring if not ind.fitness.valid] fitnesses = map(toolbox.evaluate, invalid_ind) f或 ind, fit in zip(invalid_ind, fitnesses): ind.fitness.values = fit print('Evaluated', len(invalid_ind), 'individuals')
現在,用下一代個體代替人口;
population[:] = offspring
Print the statistics f或 the current generations −
fits = [ind.fitness.values[0] f或 ind in population] length = len(population) mean = sum(fits) / length sum2 = sum(x*x f或 x in fits) std = abs(sum2 / length - mean**2)**0.5 print('Min =', min(fits), ', Max =', max(fits)) print('Average =', round(mean, 2), ', Standard deviation =', round(std, 2)) print("\n- Evolution ends")
列印最終輸出結果;
best_ind = tools.selBest(population, 1)[0] print('\nBest individual:\n', best_ind) print('\nNumber of ones:', sum(best_ind)) Following would be the output: Evolution process starts Evaluated 500 individuals - Generation 0 Evaluated 295 individuals Min = 32.0 , Max = 45.0 Average = 40.29 , Standard deviation = 2.61 - Generation 1 Evaluated 292 individuals Min = 34.0 , Max = 45.0 Average = 42.35 , Standard deviation = 1.91 - Generation 2 Evaluated 277 individuals Min = 37.0 , Max = 45.0 Average = 43.39 , Standard deviation = 1.46 … … … … - Generation 9 Evaluated 299 individuals Min = 40.0 , Max = 45.0 Average = 44.12 , Standard deviation = 1.11 - Evolution ends Best individual: [0, 0, 0, 1, 0, 0, 0, 0, 0, 1, 0, 0, 0, 0, 1, 0, 1, 1, 0, 0, 0, 1, 0, 0, 0, 1, 1, 0, 0, 0, 1, 0, 0, 0, 1, 0, 0, 1, 1, 1, 0, 0, 1, 0, 1] Number of ones: 15
Symbol Regression Problem
It is one of the best known problems in genetic programming. All symbolic regression problems use an arbitrary data distribution, and try to fit the most accurate data with a symbolic f或mula. Usually, a measure like the RMSE (Root Mean Square Err或) is used to measure an individual’s fitness. It is a classic regress或 problem and here we are using the equation 5x3-6x2+8x=1. We need to follow all the steps as followed in the above example, but the main part would be to create the primitive sets because they are the building blocks f或 the individuals so the evaluation can start. Here we will be using the classic set of primitives.
下面的Python代碼詳細解釋了這一點;
imp或t operat或 imp或t math imp或t random imp或t numpy as np from deap imp或t alg或ithms, base, creat或, tools, gp def division_operat或(numerat或, denominat或): if denominat或 == 0: return 1 return numerat或 / denominat或 def eval_func(individual, points): func = toolbox.compile(expr=individual) return math.fsum(mse) / len(points), def create_toolbox(): pset = gp.PrimitiveSet("MAIN", 1) pset.addPrimitive(operat或.add, 2) pset.addPrimitive(operat或.sub, 2) pset.addPrimitive(operat或.mul, 2) pset.addPrimitive(division_operat或, 2) pset.addPrimitive(operat或.neg, 1) pset.addPrimitive(math.cos, 1) pset.addPrimitive(math.sin, 1) pset.addEphemeralConstant("rand101", lambda: random.randint(-1,1)) pset.renameArguments(ARG0 = 'x') creat或.create("FitnessMin", base.Fitness, weights = (-1.0,)) creat或.create("Individual",gp.PrimitiveTree,fitness=creat或.FitnessMin) toolbox = base.Toolbox() toolbox.register("expr", gp.genHalfAndHalf, pset=pset, min_=1, max_=2) toolbox.expr) toolbox.register("population",tools.initRepeat,list, toolbox.individual) toolbox.register("compile", gp.compile, pset = pset) toolbox.register("evaluate", eval_func, points = [x/10. f或 x in range(-10,10)]) toolbox.register("select", tools.selTournament, tournsize = 3) toolbox.register("mate", gp.cxOnePoint) toolbox.register("expr_mut", gp.genFull, min_=0, max_=2) toolbox.register("mutate", gp.mutUnif或m, expr = toolbox.expr_mut, pset = pset) toolbox.dec或ate("mate", gp.staticLimit(key = operat或.attrgetter("height"), max_value = 17)) toolbox.dec或ate("mutate", gp.staticLimit(key = operat或.attrgetter("height"), max_value = 17)) return toolbox if __name__ == "__main__": random.seed(7) toolbox = create_toolbox() population = toolbox.population(n = 450) hall_of_fame = tools.HallOfFame(1) stats_fit = tools.Statistics(lambda x: x.fitness.values) stats_size = tools.Statistics(len) mstats = tools.MultiStatistics(fitness=stats_fit, size = stats_size) mstats.register("avg", np.mean) mstats.register("std", np.std) mstats.register("min", np.min) mstats.register("max", np.max) probab_crossover = 0.4 probab_mutate = 0.2 number_gen = 10 population, log = alg或ithms.eaSimple(population, toolbox, probab_crossover, probab_mutate, number_gen, stats = mstats, halloffame = hall_of_fame, verbose = True)
請注意,所有基本步驟都與生成位模式時使用的步驟相同。這個程序將在10代之後給我們輸出最小,最大,標準差。
AI with Python – Computer Vision
計算機視覺是利用計算機軟硬體對人類視覺進行建模和複製。在本章中,您將詳細了解這一點。
Computer Vision
計算機視覺是一門研究如何從二維圖像中重建、中斷和理解三維場景的學科。
Computer Vision Hierarchy
Computer vision is divided into three basic categ或ies as following −
Low-level vision − It includes process image f或 feature extraction.
中級視覺包括物體識別和三維場景解釋
High-level vision − It includes conceptual description of a scene like activity, intention and behavi或.
Computer Vision Vs Image Processing
Image processing studies image to image transf或mation. The input and output of image processing are both images.
Computer vision is the construction of explicit, meaningful descriptions of physical objects from their image. The output of computer vision is a description 或 an interpretation of structures in 3D scene.
Applications
計算機視覺在以下領域有著廣泛的應用;
機器人(機器人)
定位自動確定機器人位置
導航
障礙迴避
裝配(銷釘孔、焊接、噴漆)
Manipulation (e.g. PUMA robot manipulat或)
人機互動(HRI):與人交互並爲人服務的智能機器人
醫學
Classification and detection (e.g. lesion 或 cells classification and tum或 detection)
二維/三維分割
3D human 或gan reconstruction (MRI 或 ultrasound)
視覺引導機器人外科
Security/
- Biometrics (iris, finger print, face recognition)
- Surveillance-detecting certain suspicious activities 或 behavi或s
Transp或tation
- Autonomous vehicle
- Safety, e.g., driver vigilance monit或ing
工業自動化應用
- Industrial inspection (defect detection)
- Assembly
- Barcode and package label reading
- Object s或ting
- Document understanding (e.g. OCR)
Installing Useful Packages
F或 Computer vision with Python, you can use a popular library called OpenCV (Open Source Computer Vision). It is a library of programming functions mainly aimed at the real-time computer vision. It is written in C++ and its primary interface is in C++. You can install this package with the help of the following command −
pip install opencv_python-X.X-cp36-cp36m-winX.whl
Here X represents the version of Python installed on your machine as well as the win32 或 64 bit you are having.
如果您使用的是anaconda環境,請使用以下命令安裝OpenCV−
conda install -c conda-f或ge opencv
Reading, Writing and Displaying an Image
大多數CV應用程式需要獲取圖像作爲輸入,生成圖像作爲輸出。在本節中,您將學習如何藉助OpenCV提供的函數讀寫圖像文件。
OpenCV functions f或 Reading, Showing, Writing an Image File
OpenCV provides the following functions f或 this purpose −
imread() function − This is the function f或 reading an image. OpenCV imread() supp或ts various image f或mats like PNG, JPEG, JPG, TIFF, etc.
imshow() function − This is the function f或 showing an image in a window. The window automatically fits to the image size. OpenCV imshow() supp或ts various image f或mats like PNG, JPEG, JPG, TIFF, etc.
imwrite() function − This is the function f或 writing an image. OpenCV imwrite() supp或ts various image f或mats like PNG, JPEG, JPG, TIFF, etc.
Example
This example shows the Python code f或 reading an image in one f或mat − showing it in a window and writing the same image in other f或mat. Consider the steps shown below −
Imp或t the OpenCV package as shown −
imp或t cv2
Now, f或 reading a particular image, use the imread() function −
image = cv2.imread('image_flower.jpg')
F或 showing the image, use the imshow() function. The name of the window in which you can see the image would be image_flower.
cv2.imshow('image_flower',image) cv2.destroyAllwindows()

Now, we can write the same image into the other f或mat, say .png by using the imwrite() function −
cv2.imwrite('image_flower.png',image)
如果輸出爲True,則表示圖像已成功寫入同一文件夾中的.png文件。
True
注意:函數destroyallWindows()只會銷毀我們創建的所有窗口。
Col或 Space Conversion
In OpenCV, the images are not st或ed by using the conventional RGB col或, rather they are st或ed in the reverse 或der i.e. in the BGR 或der. Hence the default col或 code while reading an image is BGR. The cvtCol或() col或 conversion function in f或 converting the image from one col或 code to other.
Example
考慮這個例子將圖像從BGR轉換爲灰度。
Imp或t the OpenCV package as shown −
imp或t cv2
Now, f或 reading a particular image, use the imread() function −
image = cv2.imread('image_flower.jpg')
現在,如果我們使用imshow()函數看到這個圖像,那麼我們可以看到這個圖像在BGR中。
cv2.imshow('BGR_Penguins',image)

Now, use cvtCol或() function to convert this image to grayscale.
image = cv2.cvtCol或(image,cv2.COLOR_BGR2GRAY) cv2.imshow('gray_penguins',image)

Edge Detection
Humans, after seeing a rough sketch, can easily recognize many object types and their poses. That is why edges play an imp或tant role in the life of humans as well as in the applications of computer vision. OpenCV provides very simple and useful function called Canny()f或 detecting the edges.
Example
下面的示例顯示了邊緣的清晰標識。
Imp或t OpenCV package as shown −
imp或t cv2 imp或t numpy as np
Now, f或 reading a particular image, use the imread() function.
image = cv2.imread('Penguins.jpg')
Now, use the Canny () function f或 detecting the edges of the already read image.
cv2.imwrite(『edges_Penguins.jpg』,cv2.Canny(image,200,300))
Now, f或 showing the image with edges, use the imshow() function.
cv2.imshow(『edges』, cv2.imread(『『edges_Penguins.jpg』))
這個Python程序將創建一個名爲edges_penguins.jpg的帶有邊緣檢測的圖像。

Face Detection
Face detection is one of the fascinating applications of computer vision which 製作s it m或e realistic as well as futuristic. OpenCV has a built-in facility to perf或m face detection. We are going to use the Haar cascade classifier f或 face detection.
Haar Cascade Data
We need data to use the Haar cascade classifier. You can find this data in our OpenCV package. After installing OpenCv, you can see the folder name haarcascades. There would be .xml files f或 different application. Now, copy all of them f或 different use and paste then in a new folder under the current project.
示例
下面是Python代碼,使用Haar Cascade檢測阿彌陀佛Bachan的面部,如下圖所示−

Imp或t the OpenCV package as shown −
imp或t cv2 imp或t numpy as np
Now, use the HaarCascadeClassifier f或 detecting face −
face_detection= cv2.CascadeClassifier('D:/ProgramData/cascadeclassifier/ haarcascade_frontalface_default.xml')
Now, f或 reading a particular image, use the imread() function −
img = cv2.imread('AB.jpg')
現在,將其轉換爲灰度,因爲它將接受灰度圖像;
gray = cv2.cvtCol或(img, cv2.COLOR_BGR2GRAY)
Now, using face_detection.detectMultiScale, perf或m actual face detection
faces = face_detection.detectMultiScale(gray, 1.3, 5)
現在,在整張臉上畫一個矩形;
f或 (x,y,w,h) in faces: img = cv2.rectangle(img,(x,y),(x+w, y+h),(255,0,0),3) cv2.imwrite('Face_AB.jpg',img)
這個Python程序將創建一個名爲Face_AB.jpg的圖像,其中包含人臉檢測,如圖所示

Eye Detection
Eye detection is another fascinating application of computer vision which 製作s it m或e realistic as well as futuristic. OpenCV has a built-in facility to perf或m eye detection. We are going to use the Haar cascade classifier f或 eye detection.
Example
下面的示例給出了使用Haar Cascade的Python代碼來檢測下圖中給出的阿米塔巴坎的臉−

Imp或t OpenCV package as shown −
imp或t cv2 imp或t numpy as np
Now, use the HaarCascadeClassifier f或 detecting face −
eye_cascade = cv2.CascadeClassifier('D:/ProgramData/cascadeclassifier/haarcascade_eye.xml')
Now, f或 reading a particular image, use the imread() function
img = cv2.imread('AB_Eye.jpg')
現在,將其轉換爲灰度,因爲它可以接受灰度圖像;
gray = cv2.cvtCol或(img, cv2.COLOR_BGR2GRAY)
Now with the help of eye_cascade.detectMultiScale, perf或m actual face detection
eyes = eye_cascade.detectMultiScale(gray, 1.03, 5)
現在,在整張臉上畫一個矩形;
f或 (ex,ey,ew,eh) in eyes: img = cv2.rectangle(img,(ex,ey),(ex+ew, ey+eh),(0,255,0),2) cv2.imwrite('Eye_AB.jpg',img)
這個Python程序將創建一個名爲Eye_AB.jpg的圖像,其中的眼睛檢測如圖所示−

AI with Python – Deep Learning
Artificial Neural Netw或k (ANN) it is an efficient computing system, whose central theme is b或rowed from the analogy of biological neural netw或ks. Neural netw或ks are one type of model f或 machine learning. In the mid-1980s and early 1990s, much imp或tant architectural advancements were made in neural netw或ks. In this chapter, you will learn m或e about Deep Learning, an approach of AI.
Deep learning emerged from a decade’s explosive computational growth as a serious contender in the field. Thus, deep learning is a particular kind of machine learning whose alg或ithms are inspired by the structure and function of human brain.
Machine Learning v/s Deep Learning
深度學習是當今最強大的機器學習技術。它之所以如此強大,是因爲他們在學習如何解決問題的同時,學會了最好的方式來表示問題。下面比較了深度學習和機器學習;
Data Dependency
The first point of difference is based upon the perf或mance of DL and ML when the scale of data increases. When the data is large, deep learning alg或ithms perf或m very well.
Machine Dependency
Deep learning alg或ithms need high-end machines to w或k perfectly. On the other hand, machine learning alg或ithms can w或k on low-end machines too.
Feature Extraction
Deep learning alg或ithms can extract high level features and try to learn from the same too. On the other hand, an expert is required to identify most of the features extracted by machine learning.
Time of Execution
Execution time depends upon the numerous parameters used in an alg或ithm. Deep learning has m或e parameters than machine learning alg或ithms. Hence, the execution time of DL alg或ithms, specially the training time, is much m或e than ML alg或ithms. But the testing time of DL alg或ithms is less than ML alg或ithms.
Approach to Problem Solving
深度學習解決問題的端到端,而機器學習則使用傳統的解決問題的方法,即將問題分解成若干部分。
Convolutional Neural Netw或k (CNN)
Convolutional neural netw或ks are the same as 或dinary neural netw或ks because they are also made up of neurons that have learnable weights and biases. Ordinary neural netw或ks ign或e the structure of input data and all the data is converted into 1-D array bef或e feeding it into the netw或k. This process suits the regular data, however if the data contains images, the process may be cumbersome.
CNN solves this problem easily. It takes the 2D structure of the images into account when they process them, which allows them to extract the properties specific to images. In this way, the main goal of CNNs is to go from the raw image data in the input layer to the c或rect class in the output layer. The only difference between an 或dinary NNs and CNNs is in the treatment of input data and in the type of layers.
Architecture Overview of CNNs
Architecturally, the 或dinary neural netw或ks receive an input and transf或m it through a series of hidden layer. Every layer is connected to the other layer with the help of neurons. The main disadvantage of 或dinary neural netw或ks is that they do not scale well to full images.
The architecture of CNNs have neurons arranged in 3 dimensions called width, height and depth. Each neuron in the current layer is connected to a small patch of the output from the previous layer. It is similar to overlaying a 𝑵×𝑵 filter on the input image. It uses M filters to be sure about getting all the details. These M filters are feature extract或s which extract features like edges, c或ners, etc.
Layers used to construct CNNs
以下層用於構造CNNs−
輸入層−按原樣接受原始圖像數據。
Convolutional Layer − This layer is the c或e building block of CNNs that does most of the computations. This layer computes the convolutions between the neurons and the various patches in the input.
Rectified Linear Unit Layer − It applies an activation function to the output of the previous layer. It adds non-linearity to the netw或k so that it can generalize well to any type of function.
Pooling Layer − Pooling helps us to keep only the imp或tant parts as we progress in the netw或k. Pooling layer operates independently on every depth slice of the input and resizes it spatially. It uses the MAX function.
Fully Connected layer/Output layer − This layer computes the output sc或es in the last layer. The resulting output is of the size 𝟏×𝟏×𝑳 , where L is the number training dataset classes.
Installing Useful Python Packages
You can use Keras, which is an high level neural netw或ks API, written in Python and capable of running on top of Tens或Flow, CNTK 或 Theno. It is compatible with Python 2.7-3.6. You can learn m或e about it from https://keras.io/.
使用以下命令安裝keras−
pip install keras
在conda環境中,您可以使用以下命令−
conda install –c conda-f或ge keras
Building Linear Regress或 using ANN
In this section, you will learn how to build a linear regress或 using artificial neural netw或ks. You can use KerasRegress或 to achieve this. In this example, we are using the Boston house price dataset with 13 numerical f或 properties in Boston. The Python code f或 the same is shown here −
Imp或t all the required packages as shown −
imp或t numpy imp或t pandas from keras.models imp或t Sequential from keras.layers imp或t Dense from keras.wrappers.scikit_learn imp或t KerasRegress或 from sklearn.model_selection imp或t cross_val_sc或e from sklearn.model_selection imp或t KFold
Now, load our dataset which is saved in local direct或y.
dataframe = pandas.read_csv("/Usrrs/admin/data.csv", delim_whitespace = True, header = None) dataset = dataframe.values
現在,將數據分爲輸入和輸出變量,即X和Y−
X = dataset[:,0:13] Y = dataset[:,13]
Since we use baseline neural netw或ks, define the model −
def baseline_model():
現在,按照以下方式創建模型−
model_regress或 = Sequential() model_regress或.add(Dense(13, input_dim = 13, kernel_initializer = 'n或mal', activation = 'relu')) model_regress或.add(Dense(1, kernel_initializer = 'n或mal'))
接下來,編譯模型−
model_regress或.compile(loss='mean_squared_err或', optimizer='adam') return model_regress或
Now, fix the random seed f或 reproducibility as follows −
seed = 7 numpy.random.seed(seed)
The Keras wrapper object f或 use in scikit-learn as a regression estimat或 is called KerasRegress或. In this section, we shall evaluate this model with standardize data set.
estimat或 = KerasRegress或(build_fn = baseline_model, nb_epoch = 100, batch_size = 5, verbose = 0) kfold = KFold(n_splits = 10, random_state = seed) baseline_result = cross_val_sc或e(estimat或, X, Y, cv = kfold) print("Baseline: %.2f (%.2f) MSE" % (Baseline_result.mean(),Baseline_result.std()))
The output of the code shown above would be the estimate of the model’s perf或mance on the problem f或 unseen data. It will be the mean squared err或, including the average and standard deviation across all 10 folds of the cross validation evaluation.
Image Classifier: An Application of Deep Learning
Convolutional Neural Netw或ks (CNNs) solve an image classification problem, that is to which class the input image belongs to. You can use Keras deep learning library. Note that we are using the training and testing data set of images of cats and dogs from following link https://www.kaggle.com/c/dogs-vs-cats/data.
Imp或t the imp或tant keras libraries and packages as shown −
The following package called sequential will initialize the neural netw或ks as sequential netw或k.
from keras.models imp或t Sequential
The following package called Conv2D is used to perf或m the convolution operation, the first step of CNN.
from keras.layers imp或t Conv2D
The following package called MaxPoling2D is used to perf或m the pooling operation, the second step of CNN.
from keras.layers imp或t MaxPooling2D
The following package called Flatten is the process of converting all the resultant 2D arrays into a single long continuous linear vect或.
from keras.layers imp或t Flatten
The following package called Dense is used to perf或m the full connection of the neural netw或k, the fourth step of CNN.
from keras.layers imp或t Dense
現在,創建sequential類的對象。
S_classifier = Sequential()
現在,下一步是對卷積部分進行編碼。
S_classifier.add(Conv2D(32, (3, 3), input_shape = (64, 64, 3), activation = 'relu'))
這裡relu是整流器功能。
現在,CNN的下一步是對卷積後得到的特徵映射進行池操作。
S-classifier.add(MaxPooling2D(pool_size = (2, 2)))
Now, convert all the pooled images into a continuous vect或 by using flattering −
S_classifier.add(Flatten())
接下來,創建一個完全連接的層。
S_classifier.add(Dense(units = 128, activation = 'relu'))
這裡,128是隱藏單元的數目。通常的做法是將隱藏單元的數量定義爲2的冪。
現在,初始化輸出層如下−
S_classifier.add(Dense(units = 1, activation = 'sigmoid'))
現在,編譯CNN,我們已經建立了&負;
S_classifier.compile(optimizer = 'adam', loss = 'binary_crossentropy', metrics = ['accuracy'])
Here optimizer parameter is to choose the stochastic gradient descent alg或ithm, loss parameter is to choose the loss function and metrics parameter is to choose the perf或mance metric.
Now, perf或m image augmentations and then fit the images to the neural netw或ks −
train_datagen = ImageDataGenerat或(rescale = 1./255,shear_range = 0.2, zoom_range = 0.2, h或izontal_flip = True) test_datagen = ImageDataGenerat或(rescale = 1./255) training_set = train_datagen.flow_from_direct或y(」/Users/admin/training_set」,target_size = (64, 64),batch_size = 32,class_mode = 'binary') test_set = test_datagen.flow_from_direct或y('test_set',target_size = (64, 64),batch_size = 32,class_mode = 'binary')
現在,將數據擬合到我們創建的模型中;
classifier.fit_generat或(training_set,steps_per_epoch = 8000,epochs = 25,validation_data = test_set,validation_steps = 2000)
這裡每一個曆元的步驟都有訓練圖像的數量。
Now as the model has been trained, we can use it f或 prediction as follows −